Translate this page into:
Molecular classification based on hypoxia-associated genes and construction of the prognostic model in Chronic Obstructive Pulmonary Disease
⁎Corresponding authors. dingxianfei2023@outlook.com (Xianfei Ding), zhouqingwei2010@126.com (Qingwei Zhou)
-
Received: ,
Accepted: ,
This article was originally published by Elsevier and was migrated to Scientific Scholar after the change of Publisher.
Abstract
Hypoxia exerts a great influence on multiple cancer development while the role of hypoxia in chronic obstructive pulmonary disease (COPD) remains an enigma. Here we focused on differential expression genes (DEGs) between COPD and negative normal groups and the hypoxia-associated genes (HRGs) to explore the association between hypoxia and COPD progression. GSE54837 and GSE151052 datasets in the Gene Expression Omnibus (GEO) database were collected to perform data processing and DEGs in two datasets were analyzed (GSE54837 as the training set and GSE151052 as the validation set). 7 overlapping hypoxia-related DEGs (HRDEGs, TPD52, and RORA down-regulated vs PPP1R15A, DDIT3, DUSP1, and PDK3 up-regulated) were identified in DEGs of GSE54837 and HRGs. Also, the expression of 6 HRDEGs was investigated in GSE151052. PPP1R15A, DUSP1, and PDK3 were also up-regulated in the GSE151052 dataset. Three HRDEGs (TPD52, DDIT3, and DUSP1) were finally picked out to further analysis according to the best method. In addition, we discovered that Amygdalus Communis Vas could target DUSP1, and diclofenac was able to simultaneously target three HRDEGs. Subsequently, COPD samples were divided into three subtypes (C1, C2, and C3) according to three HRDEGs in both the training set and validation set. There was a positive relationship between TPD52 and immune features while DDIT3 and DUSP1 were negatively associated with immune features in GSE54837. The immune cells and immune features between COPD and normal groups differed from each other. C1 subtypes carried more significant immune signatures. We observed that the KEGG pathways between the three subtypes differed from each other. Interestingly, small molecules including TTNPB, arachidonyltrifluoromethane, MK.886, MS.275, and exisulind were more sensitive to COPD with severe hypoxic subtypes. Our findings revealed the association between hypoxia and COPD, which offered a novel layer for developing promising therapeutic targets in COPD treatment.
Keywords
COPD
Hypoxia
COPD hypoxia subtypes
TPD52
DDIT3
DUSP1
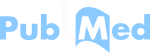
1 Introduction
Chronic obstructive pulmonary disease (COPD) is a pervasive and troublesome obstructive airway disease propelled by chronic inflammation of the respiratory tract that places an increasing burden on health care in industrialized and developing countries (Barnes, 2007; López-Campos et al., 2016). It is characterized by nonreversible airflow obstruction; primarily drags peripheral airways, that spells from air stagnation to dynamic hyperinflation and intermittent exacerbations (Tangedal et al., 2019; Fleming, 2019). COPD ails approximately 10 % of adults with age over 40 years old; and is a dominant count for admission, ranking as the third leading cause of death worldwide (Barnes et al., 2015). Although COPD typically presents as part of a multimorbidity pattern in old age; evidence has piled up to illustrate that some early life events disturb lung function, indicating that risk factors beyond those extensively reputed (examples abound: cigarette smoking and particles and gases inhaling) are glittering in the etiology of the disease (Svanes et al., 2010; Postma et al., 2015). Indeed, there is a long-standing interest in disentangling the genetic determinants from a wide range of COPD susceptibilities (Silverman, 2020). COPD is a heterogeneous disease that is subjected to genetic and environmental factors operating in a developmental climate (Decramer et al., 2012; Zhou et al., 2022). Painting a clear picture of genetic determinants in COPD allows for an unbiased evaluation of the crucial molecular determinants of disease pathobiology; which may yield encouraging insights into COPD pathogenesis (Huang et al., 2022).
COPD is frequently underdiagnosed or misdiagnosed. In general, spirometry is liable for the diagnosis of COPD; a post-bronchodilator FEV1/FVC < 0.70 acknowledges the existence of persistent airflow limitation and distinguishes patients with COPD suffering appropriate symptoms and predisposing risks (Buist et al., 2007; Singh, 2019). According to an investigation of the National Health and Nutrition Examination Survey (NHANES), more than 70 % of participants with chronic airway obstruction were not formally diagnosed with COPD (Martinez et al., 2015). The results of another analysis in five health plans have manifested that 32 % of patients with a new presumptive COPD diagnosis had been performed with spirometry to verify the detection (Han, 2007). Additionally, standardized dyspnea and symptom evaluation tools, for instance, the modified Medical Research Council (mMRC) dyspnea scale and COPD assessment test (CAT), could be capitalized on the stratification and surveillance of COPD progression (Mahler and Wells, 1988; Jones et al., 2009; Gupta et al., 2014). In the past 20 years, novel inhaled and oral medications (e.g., inhaled corticosteroids, ICS; and long-acting β2-agonists, LABAs) as well as emerging surgical and bronchoscopic procedures have been introduced for COPD treatment (Halpin, et al., 2020; Ferrera et al., 2021). Available pharmaceutical treatment for COPD can provide relief of burdensome symptoms and grind down exacerbation risk (Guo et al., 2023; Zhou et al., 2022; Lu, 2024).
In addition to medical therapy, supplementing oxygen is a momentous strategy of COPD interventions to consider in the context of severe hypoxemia. Severe hypoxia is a characteristic feature of COPD and is instrumental in inciting disease progression. Simultaneously, hypoxia is a ubiquitous attribute of a solid tumor microenvironment (TME) and is reputed as a pivotal factor in the hallmarks of cancers (Cui et al., 2022; Xu et al., 2020).
Recently, considerable research efforts are dedicated to unveiling the role of hypoxia in tumor development; however, only a few studies are in the running to dissect the function of hypoxia-related genes (HRGs) in COPD progression. A more comprehensive molecular classification and biological understanding of the HRGs in COPD could help to stratify patients into distinct phenotypes, thus contributing to the diagnosis and innovative drug development of COPD. In this study, we were posed to tap into the exact role and underlying mechanisms of HRGs in the pathological process of COPD. Notably, we interrogated the potential drug candidates targeting HRDEGs. The immune characteristics of COPD were also elucidated. Our research enlarged the current knowledge concerning the association between hypoxia and COPD.
2 Material and methods
2.1 Data acquisition
Two COPD-associated datasets, GSE151052 and GSE54837 were downloaded from the public GEO database. The HRGs were acquired from the hallmark hypoxia gene set on the hallmark website. Data were investigated by the R project. Briefly, the limma package was used to correct data background and normalization. The original probe IDs in GSE151052 and GSE54837 were transformed into gene symbols according to the probe annotation profiles. The overlapping genes between DEGs in GSE 54837 and HRGs were picked out by Venn diagrams for the subsequent analysis. We take GSE151052 as a validation cohort to further investigate the expression of these hypoxia-related DEGs (also termed as HRDEGs). The threshold of DEGs definition was P < 0.05 and |log2 FC|>1.
2.2 Identification of significant HRDEGs
Three machine learning methods including random forest (RF), generalized linear model (GLM), and support vector machine (SVM) were applied to screen out the crucial genes in distinguishing COPD from the normal sample by the DALEX package in R. Briefly, the metadata was divided into training sets and validation sets. The optimal model index of three machine learning algorithms determined the main classifier method in the following analysis. GLM was observed to produce the lowest residual in three regression models. Also, the major HRDEGs associated with COPD and normal samples were identified by the GLM method.
2.3 Prognostic model construction based on HRDEGs and validation
The important HRDEGs derived from the GLM filter were incorporated into the construction of the clinical nomograph, and the predictive accuracy of the column line graphs was verified using calibration curves and ROC curves.
2.4 The potential target drug analysis of crucial HRDEGs in TCMSP
The traditional Chinese medicine systems pharmacology (TCMSP) is an unique systemic pharmacological platform for Chinese herbal medicines where we can obtain the relationships between drugs, targets, and diseases. The database platform provides information on the identification of active ingredients, networks of compounds and drug targets, networks of related drug-target diseases, etc. We scrutinized the drug-HRDEGs networks and involved compounds of the HRDEGs. The active ingredients of drugs targeting HRDEGs were obtained separately, potential small molecule compounds were screened in the Drug Signatures Database (DSigDB, https://ngdc.cncb.ac.cn/databasecommons/database/id/4603). The molecular docking analysis was performed using autodock and vina tools to verify the relationship between compounds and targets.
2.5 COPD hypoxia subtypes analysis based on HRDEGs in GSE54837
COPD samples derived from GSE54837 were classified into three different subtypes according to three HRDEGs by non-negative matrix factorization (NMF), and the subtypes were internally validated using nearest template prediction (NTP). The expression of three HRDEGs in three subtypes was also investigated, respectively. Based on the GSE54837 dataset, the enrichment score of hypoxia flux was calculated by single-sample Gene Set Enrichment Analysis (ssGSEA) to obtain the hypoxia score. COPD subtypes samples were further classified into severe hypoxia subtypes and general hypoxia subtypes depending on the hypoxia score. ROC curves were used to evaluate the predictive performance of three HRDEGs for hypoxia subtypes.
2.6 Validation of COPD hypoxia subtypes in GSE151052
Similarly, GSE151052 was also typed using NMF to externally validate the typing results. The differences in expression of three HRDEGs and hypoxia scores among different subtypes were also analyzed. Subsequently, COPD samples originating from GSE151052 were divided into severe hypoxia subtypes and general hypoxia subtypes according to hypoxia score. ROC curves were used to assess the prediction accuracy of three HRDEGs on hypoxia subtypes.
2.7 Immune feature analysis
We also explored the expression profile of 16 immune cells and 13 immune signatures in COPD and normal groups by ssGSEA analysis. Then the correlation between three HRDEGs and immune cells and immune signatures was also probed. Next, we analyzed the differences in these immune signatures between COPD and healthy groups. Finally, we monitored the immune feature differences between COPD subtypes.
2.8 GSEA and small molecule compounds analysis between COPD hypoxia subtypes
GSEA was implemented to dissect differential pathways between hypoxia subtypes. In addition, small molecule compounds sensitive to patients with severe hypoxic subtypes identified HRDEGs were also investigated by cAMP.
3 Results
3.1 The acquisition of HRGs and DEGs in COPD
A total of 477 DEGs associated with COPD were discovered in the GSE54837 dataset (threshold as |log FC|> 1 and p-value < 0.05). The circular heatmap of DEGs between COPD and normal groups was visualized in Fig. 1A. 200 HRGs were identified by the hallmark hypoxias gene set. 6 overlapping genes were identified between DEGs and HRGs by Venn analysis (Fig. 1B). Subsequently, the gene expression profiles of 6 common HRGs were further exemplified in GSE54837. TPD52 and RORA were down-regulated in COPD samples while PPP1R15A, DUSP1, PDK3, and DDIT3 were up-regulated in COPD samples (Fig. 1C). Similarly, we discovered that PPP1R15A, DUSP1, and PDK3 were also up-regulated in the GES151052 dataset (Fig. 1D).The acquisition of HRGs and DEGs in COPD. (A) The circular heatmap of DEGs in COPD groups in comparison with normal groups. (B) The Venn result of DEGs and HRGs. (C-D) The expression profile of 6 overlapping genes in GSE54837 and GSE151052.
3.2 The identification of three imperative HRDEGs by machine learning method
We used RF, GLM, and SVM, three machine learning methods to figure out the HRGs that contributed greatly to COPD. The residual of the GLM method was the lowest of the three algorithms (Fig. 2A and 2B). The ROC analysis results showed that the AUC value of the GLM method was higher than RF and SAM (Fig. 2C). These findings demonstrated that the GLM algorithm performed excellently in the construction of the prognostic model of COPD. Results suggested that the importance of 6 overlapping HRDEGs varied according to three methods and they were different from each other (Fig. 2D). According to the GLM investigation result, the top three HRDEGs (TPD52, DDIT3, and DUSP1) were figured out to further analysis. ROC analysis indicated that TPD52 obtained the highest AUC value while DDIT3 obtained the lowest diagnostic value in COPD (Fig. 2E). The clinical nomogram of the three top HRDEGs was shown in Fig. 2F. Three HRDEGs could well distinguish COPD from normal samples. The calibration plot and ROC curves validated their good predictive power in COPD (Fig. 2G and 2H).Identification of three crucial HRDEGs. (A) The reverse cumulative distribution of residual of three learning methods. (B) the boxes plot of residual for RF, GLM, and SVM. (C) The ROC curve of residual for RF, GLM, and SVM. (D) Top 6 HRDEGs lists by RF, GLM, and SVM screening. (E) The ROC curve of three HRDEGs identified by GLM. (F) The nomogram of three top HRDEGs. (G-H) The calibration plot and ROC analysis of prognosis nomogram.
3.3 Potential drugs candidate of three HRDEGs
The screening of the TCMSP herbal database hinted at a natural compound targeting DUSP1, 17-beta-estradiol, which was the active ingredient of Amygdalus Communis Vas (also named bitter almond) (Fig. 3A). The protein product of DUSP1 could bind with 17-beta-estradiol by molecular docking analysis (Fig. 3B), indicating that Amygdalus Communis Vas could recognize DUSP1. We retained the top ten compounds of the combined score of the three HRGs in the DSigDB database (Fig. 3C). Molecular dock analysis found that diclofenac (top 1 compound) was able to simultaneously target TPD52 (Fig. 3D), DDIT3 (Fig. 3E), and DUSP1 (Fig. 3F). These findings revealed that diclofenac may be a candidate drug for treating hypoxia associated with COPD.The drug-HRDEGs interaction analysis. (A) The interaction between 17-beta- estradiol and DUSP1. (B) Molecular dock results of DUSP1 with 17-beta-estradiol. (C) DSigDB database showed the top ten compounds of the combined score of three HRDEGs. (D-F) Molecular dock results of three HRDEGs and diclofenac.
3.4 COPD subtypes analysis based on three HDRGs in GSE54837
The COPD samples were clustered by NMF, and it was found that the sample typing did the best performance at r = 3 (Fig. 4A). The matrix heat map also showed that COPD samples could be divided into three subtypes (named C1, C2, and C3) (Fig. 4B). The classification was verified by the NTP algorithm according to the top 100 upregulated genes of each subtype. The samples could also be broken down into three subtypes (Fig. 4C). Calibration plots displayed that NTP classification and NMF classification had a good correlation (r = 0.64, Fig. 4D). Among the three HDRGs, TPD52 was highly expressed in the C1 subtype (Fig. 4E), DDIT3 was predominantly elevated in the C3 subtype (Fig. 4F), and DUSP1 showed significant upregulation in the C2 subtype (Fig. 4G). Hypoxia score was higher in C2 and C3 subtypes than C1 subtype (Fig. 4H). Therefore, we defined C2 and C3 subtypes as the severe hypoxia subtype and C1 as the general hypoxia subtype. All three HDRGs had excellent predictive performance in predicting both hypoxia subtypes, with TPD52 being the most accurate (Fig. 4I).Identification of COPD subtypes based on three HRDEGs (A) The sample typing result had a good performance of NMF at r = 3. (B) The heatmap of three COPD subtypes by NMF. (C) The heatmap of three subtypes was validated by the NTP algorithm. (D) The correlation analysis between NTP and NMF typing. (E-G) The expression of three HRDEGs in three COPD subtypes. (H) Hypoxia flux enrichment scores of three subtypes. (I) ROC curves of three HRDEGs in predicting COPD hypoxia subtypes.
3.5 The endorsement of COPD typing based on three HRDEGs in GSE151052
We investigated the expression level of three HRDEGs in GSE151052 and COPD samples could be also classified into three subtypes (C1, C2, and C3) by the NMF typing method (Fig. 5A). Among the three HDRGs, TPD52 was highly expressed in C2 (Fig. 5B), DDIT3 was with highest expression in C1 (Fig. 5C), and DUSP1 expression was highest in C3 (Fig. 5D). The hypoxia score was higher in C1 and C3 subtypes (Fig. 5E). Similarly, we defined C1 and C3 as the severe hypoxia subtype and C2 as the general hypoxia subtype. TPD52 and DUSP1 predicted these two hypoxic subtypes well, and TPD52 was the most accurate prediction (Fig. 5F). This is essentially the same situation as in GSE54837.Validation of COPD hypoxia typing in GSE15102 (A)The heatmap of three subtypes identified by three HRDEGs in GSE151052 via NMF. (B-D) The expression of three HRDEGs in three subtypes in GSE151052. (E) Hypoxia flux enrichment scores of three subtypes. (F) ROC curves of three HRDEGs in distinguishing COPD hypoxia subtypes.
3.6 Immune characteristics analysis in COPD based on GSE54837
Among the three HRDEGs, TPD52 was positively correlated with immune features compared to DDIT3 and DUSP1, while DDIT3 and DUSP1 were negatively correlated with immune features (Fig. 6A). Relative to healthy individuals, immune features such as APC co-inhibition, type I IFN response, type II IFN response, and mast cells were more highly expressed in COPD (Fig. 6B). Immune cells such as B cells, CD8+ T cells, neutrophils, TIL, and immune signatures including APC co-stimulation, inflammation-promoting, MHC class I, type II IFN response, and other immune signatures differed in three subtypes (Fig. 6C). We found that most of the immune signatures with significant expression were highly expressed in the C1 subtype of COPD (Fig. 6D). It was concluded that patients with the general hypoxic subtype had better immunogenicity.Immune cell and immune feature analysis of three HRDEGs in COPD dataset GSE54837. (A) The correlation between three HRDEGs and 16 immune cells and 13 immune signatures. (B) The expression profile of 16 immune cells and 13 immune signatures in COPD and normal groups. (C) The expression profile of 16 immune cells and 13 immune signatures in three subtypes. (D) Immune feature landscape in COPD subtypes.
3.7 KEGG analysis in COPD hypoxia subtypes
We investigated the pathways enrichment between three COPD subtypes. Results indicated that the adrenergic angiotensin system and sulfur metabolism pathways were downregulated in C1 while the cytosolic DNA sensing pathway was downregulated in C2. Glycosaminoglycan biosynthesis keratan sulfate was down-regulated in C3 (Fig. 7A). Ribosome and protein export were up-regulated in C1 while porphyrin and chlorophyll metabolism pathways were up-regulated in C2 while non-homologous end- joining was up-regulated in C3 (Fig. 7B). This research uncovered that COPD with different hypoxia contexts exhibited metabolism pathways.KEGG analysis in COPD hypoxia subtypes. (A-B) The KEGG enrichment results for COPD subtypes in the GSE54837 and GSE151052 datasets.
3.8 Compounds sensitive to COPD with severe hypoxic context
The crucial metabolism signal, cAMP, was used to explore the association between COPD subtypes and small molecule compounds. TTNPB, arachidonyltrifluoromethane, MK.886, MS.275, and exisulind were found to be sensitive to COPD patients with severe hypoxic subtypes (Fig. 8). This could guide the treatment of patients with severe hypoxic subtypes of COPD.Compounds sensitive to COPD with severe hypoxia.
4 Discussion
Despite the yawning clout of cigarette smoking, genetic factors are also essential determinants of COPD. Indeed, numerous genomic regions that affect COPD susceptibility have been defined during the genome-wide correlation analysis, of which a quintessential example would be alpha-1 antitrypsin deficiency, a pervasive single-gene disorder among Northern Europeans and North Americans (Chiuchiolo and Crystal, 2016). Simultaneously, some genomic regions correlated with COPD-related phenotypes, for instance, quantitative measures of emphysema, have been identified (Silverman, 2018; Chen et al., 2019). Disentangling the functional variants and core genes within these linked regions is still riddled with challenges. The current study was designed to come to grips with the role of HRGs in COPD progression and their diagnostic value (He et al., 2019; Peng et al., 2022).
We first gleaned 477 DEGs between the healthy and COPD samples of the GSE54837 dataset. Further, 200 HRGs were obtained and 6 overlapping HRDEGs (TPD52, RORA, PPP1R15A, DDIT3, DUSP1, and PDK3) were screened between the DEGs and HRGs. Interestingly, the expression levels of TPD52 and RORA were tail off while counterparts of PPP1R15A, DDIT3, DUSP1, and PDK3 exhibited a mounting trajectory in COPD. The trend of PPP1R15A, DUSP1 and PDK3 were also observed in the validated set (GSE151052). PPP1R15A, serving as a stress-induced regulatory subunit, was sufficient to negatively modulate eIF2α dephosphorylation (Hölzer et al., 2016). Phosphorylation of eIF2α is an evolutionarily conserved and critical cellular defense system that can counteract multiple forms of stress (Carrara et al., 2017). Studies have shown that PPP1R15A was sinking in lung fibroblasts of patients with idiopathic pulmonary fibrosis (IPF; an unrelenting; chronic, and progressive lung disease that could be triggered by smoking) (Monkley et al., 2021; Richeldi et al., 2017). TGF-β-educated fibroblasts further shrunk PPPIR15A. The absence of PPPIR15A or its pharmacological handcuff aggravated experimental lung fibrosis. Whether such an effect is thrown on COPD remains currently unclear.
Three machine learning algorithms (GLM, RF, and SVM) demonstrated the varied clout of 6 HRDEGs in COPD progression; and, consequent to that, 3 paramount HRDEGs (TPD52, DDIT3, and DUSP1) were finally identified based on GLM analysis as it presented the largest area under ROC curve. Taken as a whole, the top three key HRDEGs, albeit with distinct predictive probability, performed well in distinguishing patients with COPD. The tumor protein D52 (TPD52) family was generally interpreted as a crucial driver in the proliferation and metastasis of a wide range of cancers (Fan et al., 2021; Byrne et al., 2005). TPD52L2, a member belonging to the TPD52-like protein family, was overexpressed and associated with unfavorable prognosis in a variety of cancers, including lung adenocarcinoma, glioblastoma, and prostate cancer (Zhong et al., 2021; Qiang et al., 2018; Ren et al., 2017). However, studies centered around TPD52 in COPD are still scarce. Our study gives a window to decode the role of TPD52 in COPD progression and its potential in clinical diagnosis.
In recent years, a large number of studies concerning traditional herbal medicine have been published, and many attractive applications have been explored (Li and Weng, 2017; Zhao et al., 2019). Having a series of surfing among the TCMSP herbal medicine, it was observed that, 17-β- estradiol, the biologically active component of Amygdalus Communis Vas, could bind to DUSP1. Low-expressed DUSP1 galvanized alternation later expression pattern of genes engaged in certain biological pathways, including angiogenesis, cellular communication, and tyrosine-kinase receptor activity (Moncho-Amor et al., 2011). Such alternation was largely boiled down to the administration of c-Jun-N-terminal kinase and/or p38 activity by DUSP1 (Hao et al., 2015). It has been reported that upregulation of DUSP1 abundance and consequently altered patterns of JNK and p38 activities are strongly associated with NSCLC initiation, invasion, and metastasis (Moncho-Amor et al., 2011). A proportion of patients with COPD progressed rapidly to SCLC, despite the molecular mechanisms linking COPD with lung cancer development being far from clear (Cho et al., 2018; Xiao et al., 2017). Overall, we identified a novel kind of herbal medicine that can target DUSP1, thus manipulating its expression levels. Moreover, we found that diclofenac, with the highest combined score, could act on TPD52, DDIT3, and DUSP1.
Given the importance of HRGs in the progression of COPD, it could be advantageous to develop key HRGs as biomarkers that contribute to distinguishing COPD patients. We defined three hypoxia subtypes (namely C1, C2, and C3) by consensus clustering based on the expression landscape of three HRDEGs. In GSE54837, TPD52, DDIT3, and DUSP1 showed an overexpression tendency in the C1, C3, and C2 subtypes, respectively. According to the hypoxia score, we nominated C2 and C3 as the severe hypoxia subgroup and C1 as the normal hypoxia subgroup. The three pivotal HRDEGs, in particular, TPD52, performed well in stratifying COPD patients into the two subgroups with varying degrees of hypoxia. Such characteristics of the HRDEGs exhibited a high diagnostic value in terms of COPD. In the validated set GSE151052, we found that the expression of TPD52 was upregulated in C2, and DDIT3 was overexpressed in C3. We defined C1 and C3 as the severe hypoxia subgroup and C2 as the normal hypoxia subgroup. Within this framework, TPD52 also showed considerable precision in identifying the specific hypoxia subgroups among COPD patients, reinforcing its utility in the molecular stratification of the disease based on hypoxia status.
There are, to date, very limited studies comparing the pathology between COPD and other causes of disease other than cigarette smoking (e.g., immunopathology), to this end, we were in the running to tackle this issue through ascertaining immune landscape among different subtypes (Birring et al., 2002; Rivera et al., 2008). Interestingly, TPD52 exhibited a positive correlation with immune characteristics, while DDIT3 and DUSP1 displayed a negative association. Immune features like APC co-inhibition, type-I IFN response, type II IFN response, and mast cells contributed to the identification just mentioned. These could be employed to estimate the immunity of patients with COPD. Alternatively, hypoxia subtypes could be cooperated with the immune features to make a comprehensive consideration of the patient’s condition. Such a combination might not only identify patients who could benefit from immunotherapy but also cast light on predicting the prognosis of COPD patients.
Enrichment analysis demonstrated the difference in pathways among the three subtypes, further confirming the specific immune landscape in them. Aside from these, we also explored some agents, for example, TTNPB, MK.886, and MS.275, that were engineered to alleviate the progression of COPD patients in the severe subtype.
5 Conclusion
In conclusion, our study emphasized the role of HRGs in COPD progression and identified three COPD hypoxia subtypes based on predominant HRDEGs (TPD52, DUSP1, and DDIT3). The immune characteristics of COPD hypoxia subtypes differed from each other. These observations may provide clues for the diagnosis and treatment of patients with COPD.
Availability of data and materials: The dataset supporting the conclusions of this article is included within the article.
Funding
None.
CRediT authorship contribution statement
Zhongshuai Fu, Dongsheng Song conceived the study and wrote the article. Qingrong Cui, Danbo Li, Beilei Wang contributed to data interpretation. Qingwei Zhou and Xianfei Ding revised the article.
Acknowledgements
None.
Declaration of competing interest
The authors declare that they have no known competing financial interests or personal relationships that could have appeared to influence the work reported in this paper.
References
- Chronic obstructive pulmonary disease: a growing but neglected global epidemic. PLoS Med.. 2007;4(5):e112.
- [Google Scholar]
- Clinical, radiologic, and induced sputum features of chronic obstructive pulmonary disease in nonsmokers: a descriptive study. Am. J. Respir. Crit. Care Med.. 2002;166(8):1078-1083.
- [Google Scholar]
- International variation in the prevalence of COPD (the BOLD Study): a population-based prevalence study. Lancet. 2007;370(9589):741-750.
- [Google Scholar]
- Tumor protein D52 (TPD52) is overexpressed and a gene amplification target in ovarian cancer. Int. J. Cancer. 2005;117(6):1049-1054.
- [Google Scholar]
- Decoding the selectivity of eIF2α holophosphatases and PPP1R15A inhibitors. Nat. Struct. Mol. Biol.. 2017;24(9):708-716.
- [Google Scholar]
- Introduction of exogenous wild-type p53 mediates the regulation of oncoprotein 18/stathmin signaling via nuclear factor-κB in non-small cell lung cancer NCI-H1299 cells. Oncol. Rep.. 2019;41(3):2051-2059.
- [Google Scholar]
- Gene therapy for alpha-1 antitrypsin deficiency lung disease. Ann. Am. Thorac. Soc.. 2016;13(Supplement 4):S352-S369.
- [Google Scholar]
- Prognostic implication of FEV1/FVC ratio for limited-stage small cell lung cancer. J. Thorac. Dis.. 2018;10(3):1797.
- [Google Scholar]
- MiR-29a-3p improves acute lung injury by reducing alveolar epithelial cell PANoptosis. Aging Dis.. 2022;13(3):899.
- [Google Scholar]
- Decramer, M., W. Janssens, and M. Miravitlles, Chronic obstructive pulmonary disease. Lancet [Internet]. 2012, Elsevier Ltd.
- Acetylation-dependent regulation of TPD52 isoform 1 modulates chaperone-mediated autophagy in prostate cancer. Autophagy. 2021;17(12):4386-4400.
- [Google Scholar]
- Advances in chronic obstructive pulmonary disease. Annu. Rev. Med.. 2021;72:119-134.
- [Google Scholar]
- Quantitative assessment of mucociliary clearance in smokers with mild-to-moderate chronic obstructive pulmonary disease and chronic bronchitis from planar radionuclide imaging using the change in penetration index. J. Aerosol Med. Pulm. Drug Deliv.. 2019;32(4):175-188.
- [Google Scholar]
- LncRNA FAS-AS1 upregulated by its genetic variation rs6586163 promotes cell apoptosis in nasopharyngeal carcinoma through regulating mitochondria function and Fas splicing. Sci. Rep.. 2023;13(1):8218.
- [Google Scholar]
- The COPD assessment test: a systematic review. Eur. Respir. J.. 2014;44(4):873-884.
- [Google Scholar]
- Halpin, D.M., et al., Global initiative for the diagnosis, management, and prevention of chronic obstructive lung disease. The 2020 GOLD science committee report on COVID-19 and chronic obstructive pulmonary disease. American journal of respiratory and critical care medicine, 2021. 203(1): p. 24-36.
- Disruption of a regulatory loop between DUSP1 and p53 contributes to hepatocellular carcinoma development and progression. J. Hepatol.. 2015;62(6):1278-1286.
- [Google Scholar]
- Frequency of depression-related symptoms in caregivers of patients with silicosis. J. Healthcare Eng.. 2019;2019
- [Google Scholar]
- Differential transcriptional responses to Ebola and Marburg virus infection in bat and human cells. Sci. Rep.. 2016;6(1):34589.
- [Google Scholar]
- SLNL: a novel method for gene selection and phenotype classification. Int. J. Intell. Syst.. 2022;37(9):6283-6304.
- [Google Scholar]
- Development and first validation of the COPD Assessment Test. Eur. Respir. J.. 2009;34(3):648-654.
- [Google Scholar]
- Demystifying traditional herbal medicine with modern approach. Nat. Plants. 2017;3(8):1-7.
- [Google Scholar]
- Surgical instrument posture estimation and tracking based on LSTM. ICT Express 2024
- [Google Scholar]
- Undiagnosed obstructive lung disease in the United States. Associated factors and long-term mortality. Ann. Am. Thorac. Soc.. 2015;12(12):1788-1795.
- [Google Scholar]
- DUSP1/MKP1 promotes angiogenesis, invasion and metastasis in non-small-cell lung cancer. Oncogene. 2011;30(6):668-678.
- [Google Scholar]
- Sensitization of the UPR by loss of PPP1R15A promotes fibrosis and senescence in IPF. Sci. Rep.. 2021;11(1):21584.
- [Google Scholar]
- Prognostic factors in stage IV colorectal cancer patients with resection of liver and/or pulmonary metastases: a population-based cohort study. Front. Oncol.. 2022;12:850937
- [Google Scholar]
- Risk factors and early origins of chronic obstructive pulmonary disease. Lancet. 2015;385(9971):899-909.
- [Google Scholar]
- TPD52L2 impacts proliferation, invasiveness and apoptosis of glioblastoma cells via modulation of wnt/β-catenin/snail signaling. Carcinogenesis. 2018;39(2):214-224.
- [Google Scholar]
- Increased expression of tumor protein D54 is associated with clinical progression and poor prognosis in patients with prostate cancer. Oncol. Lett.. 2017;14(6):7739-7744.
- [Google Scholar]
- Comparison of lung morphology in COPD secondary to cigarette and biomass smoke. Int. J. Tuberc. Lung Dis.. 2008;12(8):972-977.
- [Google Scholar]
- Applying functional genomics to chronic obstructive pulmonary disease. Ann. Am. Thorac. Soc.. 2018;15(Supplement 4):S239-S242.
- [Google Scholar]
- Global strategy for the diagnosis, management, and prevention of chronic obstructive lung disease: the GOLD science committee report 2019. Eur. Respir. J.. 2019;53(5)
- [Google Scholar]
- Early life origins of chronic obstructive pulmonary disease. Thorax. 2010;65(1):14-20.
- [Google Scholar]
- Sputum microbiota and inflammation at stable state and during exacerbations in a cohort of chronic obstructive pulmonary disease (COPD) patients. PLoS One. 2019;14(9):e0222449.
- [Google Scholar]
- Integrative analysis of genomic sequencing data reveals higher prevalence of LRP1B mutations in lung adenocarcinoma patients with COPD. Sci. Rep.. 2017;7(1):2121.
- [Google Scholar]
- Identification of key genes in ruptured atherosclerotic plaques by weighted gene correlation network analysis. Sci. Rep.. 2020;10(1):10847.
- [Google Scholar]
- In vitro neutralization of autocrine IL-10 affects Op18/stathmin signaling in non-small cell lung cancer cells. Oncol. Rep.. 2019;41(1):501-511.
- [Google Scholar]
- TPD52L2 is a prognostic biomarker and correlated with immune infiltration in lung adenocarcinoma. Front. Pharmacol.. 2021;12:728420
- [Google Scholar]
- Regulatory roles of three miRNAs on allergen mRNA expression in Tyrophagus putrescentiae. Allergy. 2022;77(2):469-482.
- [Google Scholar]
- Dermatophagoides pteronyssinus allergen Der p 22: Cloning, expression, IgE-binding in asthmatic children, and immunogenicity. Pediatr. Allergy Immunol.. 2022;33(8):e13835.
- [Google Scholar]