Translate this page into:
Investigation of “Microbial-mitochondrial” cross-talk to explore the compatibility mechanism of Xiaojianzhong Tang against chronic atrophic gastritis rats
* Corresponding author: E-mail address: yuetaoliu@sxu.edu.cn (Y. Liu)
-
Received: ,
Accepted: ,
Abstract
Chronic atrophic gastritis (CAG) is one of the common digestive disorders. Xiaojianzhong Tang (XJZ) is a classic traditional Chinese medicine prescription (TCMP) used for the treatment of chronic atrophic gastritis, but its compatibility mechanism has not been fully clarified. This study integrated 16S rRNA sequencing, metabolomics, and molecular docking to explore the role of “Microbial mitochondrial” cross-talk in the treatment of CAG with XJZ and its subtracted formulas. CAG could cause disorders in gastric mucosal microbiota and gastric tissue metabolism. 17 gastric tissue differential metabolites were directly or indirectly derived from microbes and were associated with mitochondrial function. Further research had found that CAG could also cause mitochondrial metabolic disorders, with 18 mitochondrial metabolites involved in purine metabolism, glutathione metabolism, biosynthesis of various other secondary metabolites, nicotinate and nicotinamide metabolism, and aminoacyl-tRNA biosynthesis. XJZ showed the strongest effect, followed by XJZ-C (XJZ without “complement drug”) and XJZ-A (XJZ without “assistant drug”), and XJZ-P (XJZ without “principal drug”) the least. Protein-protein interaction (PPI) and molecular docking showed that mitochondrial metabolic enzymes could be potentially affected by microbial metabolites. In conclusion, there was a cross-talk between gastric mucosal microbes and mitochondria. In the treatment of CAG by XJZ, “principal drug” was the most important, and “assistant drug” and “complement drug” could assist the “principal drug”.
Keywords
Chronic atrophic gastritis
Compatibility mechanism
Gastric mucosal microbiota
Metabolomics
“Microbial-mitochondrial” cross-talk
Xiaojianzhong Tang
1. Introduction
Chronic atrophic gastritis (CAG) is one of the common gastric diseases, with Helicobacter pylori and bile reflux being its main causes [1]. The pathological features of CAG mainly include gastric mucosal thinning, gastric mucosal gland atrophy, elevated gastric juice pH, and decreased pepsin activity (PA) [2,3]. In clinical practice, anti-Helicobacter pylori drugs, anti-acid drugs and mucosal protective drugs are the main treatment methods targeting these complex pathogeneses. Although they can alleviate the clinical symptoms of patients to a certain extent, the long-term effect is far from satisfactory. Therefore, it is urgent to develop innovative drugs that can treat CAG with good efficacy, few side effects, and low cost.
As a science with long history in China, traditional Chinese medicine prescriptions (TCMPs) play important roles in the treatment of diseases, where their compatibility is considered as their key rule in the theory of TCMPs [4]. In the ancient medical classic “Huangdi Neijing”, there is a kind of compatibility thought called “principal,” “assistant,” “mediator,” and “complement”. In this theory, “principal drug” represents a basic component of the prescription, and its main role is to target the cause of the disease or the main syndrome. “Assistant drug” and “Complement drug” refer to the components that can enhance the efficacy of “principal drug”. However, unclear chemical components, unverified functional targets and ambiguous molecular mechanisms have hindered the widespread use of TCMP worldwide [5]. In order to explore their scientific connotations, in-depth research is needed.
Xiaojianzhong Tang (XJZ) is derived from Zhang Zhongjing’s classic work “Synopsis of the Golden Chamber”. It consists of six Traditional Chinese medicine (TCM) herbs, of which Saccharum granorum (Yitang, YT) is its “principal drug,” Cinnamomum cassia Presl (Guizhi, GZ) and Paeonia lactiflora Pall (Baishao, BS) are its “assistant drugs,” and Ziziphus jujuba Mill (Dazao, DZ), Zingiber officinale Rosc (Shengjiang, SJ) and Glycyrrhiza uralensis Fisch (Gancao, GC) are its “complement drugs.” Studies have shown that XJZ has a good effect on the treatment of CAG by regulating intestinal microbiota and bile acid metabolism [6], but its mechanism of action remains to be studied. It is worth noting that there is no research on their compatibility mechanism in the treatment of CAG.
Due to the strong acidic environment of the stomach and the characteristics of the digestive peristalsis of the stomach, it had always been considered that the stomach was a sterile organ without microbiota. Until 1982 when the discovery of Helicobacter pylori changed people ‘s views [7]. With the development of high-throughput sequencing and bioinformatics technology, it had been found that Helicobacter pylori was not the only microbe that survives in the stomach, and that there were other types of microbes. Compared with intestinal microbiota, gastric microbiota had the characteristics of low density, low specificity, poor stability, and high volatility [8]. The sequencing results showed that the microbes in the stomach included Firmicutes, Bacteroidetes, Proteobacteria, Actinobacteria, and Fusobacteria phyla, which were significantly different from the microbial sequence sets of human mouth and esophagus, indicating that the human stomach might be an independent microbial ecosystem [9]. Gastric microbiota could maintain endocrine balance, immune regulation, and promote digestion and absorption, etc. Therefore, maintaining gastric microecological balance is of great significance to gastric health. When the stomach is affected by bile reflux and Helicobacter pylori infection, it will destroy the gastric environment and physiological structure, and cause changes in the structure of the microbiota, and then lead to the formation and development of diseases. Therefore, paying attention to gastric mucosal microbiota can provide new ideas for the mechanism of XJZ and its subtracted formulas in the treatment of CAG.
Mitochondria are widely distributed organelles in organisms, which can not only maintain cellular bioenergetics by producing adenosine triphosphate (ATP), but also synthesize metabolites, produce reactive oxygen species (ROS), transmit immune signals and mediate apoptosis [10]. Mitochondrial dysfunction has been observed in a variety of diseases, including obesity, inflammation, cancer, and neurodegenerative diseases [11,12]. There is also a strong link between the occurrence of CAG and mitochondrial dysfunction [13,14]. Microbes can directly or indirectly regulate mitochondrial function through metabolites, such as short chain fatty acids (SCFAs), H2S, and NO, affect mitochondria-related energy metabolism, regulate the production of ROS, and regulate the immunity of mitochondria and even the whole body [15,16]. Xu found that XJZ could regulate microbes and metabolites in gastric juice, and the regulation of some metabolites was mediated by microbes [17]. YT, GZ, SJ, BS, GC and GZ all individually modulated microbes and metabolites with different effects [18-23]. Therefore, paying attention to the cross-talk between gastric mucosal microbes and mitochondria can provide new ideas for the mechanism of XJZ and its subtracted formulas in the treatment of CAG.
Metabolomics could monitor the metabolic changes in response to external stimulation, which was a branch of system biology [24]. Metabolomics was unique and significant in mining the functional changes of small molecule metabolites as a whole, which was consistent with the scientific connotation of TCMP. Meanwhile, metabolomics also showed potential insights into the metabolic alterations of intestinal microbiota, which was helpful to detect the unexpected results. MetOrigin was a bioinformatics tool that identifies which bacteria and how they participated in certain metabolic reactions, helping us understand where the metabolites come from: the host, the bacteria, or both [25]. It could quickly identify microbial-related metabolites and analyze their metabolic functions. The introduction of MetOrigin into metabolomics analysis enabled us to more accurately analyze the source and function of metabolites and explore the compatibility mechanism of TCMP.
In this study, the CAG rat model was used to evaluate the pharmacological effects of XJZ and XJZ without “principal drug” (XJZ-P), XJZ without “assistant drug” (XJZ-A), and XJZ without “complement drug” (XJZ-C). At the same time, combined with metabolomics, 16S rRNA sequencing and MetOrigin, the compatibility mechanism of XJZ in the treatment of CAG was explored from the changes in intestinal microbiota and metabolites.
2. Material and Methods
2.1. Reagents and materials
Sodium deoxycholate was purchased from Beijing Aoboxing Biotechnology Co., Ltd. (Beijing, China). Ammonia was purchased from Tianjin Damao Chemical Reagent Factory (Tianjin, China). Teprenone was purchased from Self-Defense Pharmaceutical Co., Ltd. (Nanjing, Jiangsu, China). Saline was purchased from North China Pharmaceutical Co., Ltd. (Shijiazhuang, Hebei). Neutral formalin fixative (10%) was purchased from Beijing Solebold Technology Co., Ltd. The pepsin assay kit was purchased from Nanjing Jiancheng Bioengineering Institute (Nanjing, Jiangsu, China). Mass spectrometry grade -methanol, formic acid, and acetonitrile were purchased from Thermo Fisher Co., Ltd. (Waltham, MA, USA). All the chemical reagents applied in this experiment were of analytical rank.
GZ, BS, DZ, and GC were purchased from Tongrentang Pharmacy (Taiyuan, Shanxi, China). YT was purchased from Lingui Tianxiang Food Raw Material Co., Ltd. (Guilin, Guangxi, China). SJ was purchased from Xuxi farmers ‘ market (Taiyuan, Shanxi, China). All the above medicinal materials were identified by Professor Xuemei Qin of the Modern Research Center of TCM of Shanxi University, and all the herbs were in accordance with the “Pharmacopoeia of the People ‘s Republic of China 2020” [5]. The samples were preserved in the cold storage of the Center for Modern Research of TCM, Shanxi University.
2.2. Preparation of XJZ and its subtracted formulas
Previous studies were screened for the most effective dose of XJZ [17]: GZ (18 g), GC (12 g), DZ (30 g), BS (36 g), SJ (18 g), and YT (60 g). First, 1740 mL (10 times the quality of medicinal material) of water was added to the medicinal material, soaked for 1 hr, and then heated and refluxed for 1 hr. The filtrate was collected and the above extration was repeated again. The two filtrates were combined, and the combination was initially concentrated under reduced pressure, and then YT was added to dissolve. XJZ-P, XJZ-A, and XJZ-C were prepared by subtracting the “principal drug (YT)”, “assistant drug (GZ and BS),” and “complement drug (DZ, SJ and GC)” from XJZ, respectively. The composition of XJZ and its subtracted formulas have been shown in Table S1. Additionally, the chemical analysis was performed by Ultra-High Performance Liquid Chromatography Quadrupole/Time-of-Flight Mass Spectrometry (UHPLC-Q/TOF-MS), where a total of 785 components were characterized in XJZ including 385 compounds from GC, 99 compounds from GZ, 89 compounds from DZ, 151 compounds from BS, and 64 compounds from SJ [26]. The typical chromatograms of XJZ have been showed in Figure S1.
2.3. Animal experiment
2.3.1. Animal grouping, modeling and administration
All experiments were conducted according to the National Institutes of Health published “Laboratory Animal Care and Laboratory Animal Use Principles” (NIH Publication, 8th Edition, 2011). All rat experimental procedures were approved by the Animal Ethics Committee of Shanxi University (SXULL2021029). The animals in the experiment were purchased from Beijing Vital River Laboratory Animal Technology Co., Ltd. A total of 42 Specific Pathogen Free (SPF) male Sprague Dawley (SD) rats (180 g-200 g) were purchased for feeding and subsequent experiments in the SPF animal room. Rats were raised in separate cages, 3 rats in each cage. Feeding conditions were standard laboratory environment (temperature: 25 ± 2°C; relative humidity: 60% ± 5%; day/night cycle: 12 hrs).
Before the start of the experiment, a week of adaptive feeding was carried out and all the rats drank and ate freely. After the adaptive feeding, all rats were randomly divided into 7 groups according to body weight, 6 rats in each group, including control group (control), model group (model), positive drug group (Pos), XJZ group, XJZ-P group, XJZ-A group, and XJZ-C group. Rats in the control group were fed with a standard diet and free to drink water. The other rats were subjected to the replication of the CAG model. The replication details were performed as follows: two days of foot food and one day of fasting, ammonia (0.1%) and sodium deoxycholate solution (20 mmol/L) as drinking water were used alternately every day. The modeling lasted for 10 weeks, and fatigue swimming was performed once a week in the last 4 weeks. In the last four weeks, XJZ group, subtracted formula groups and Pos group (teprenone, 130 mg/kg/d) were given the corresponding drugs by gavage. Based on the conversion rate between rats and humans (6.17:1) [27], the daily intake of each prescription in rats were calculated and has been showed in Table S1. Food intake was measured during the experiment, and body weight was recorded every 6 days.
2.3.2. Sample collection
After the last weighing, these experimental animals were fasted for 24 hrs with free drinking water. After anesthesia with ether, the pylorus of the stomach was ligated and the abdominal cavity was sutured. After 3 hrs, the rats were anesthetized, the cardia was ligated, and blood samples were collected from the abdominal aorta. The whole stomach was removed and gastric juice was collected. After centrifugation at 5000 rpm for 10 mins at 4°C, the supernatant was stored and its pH and PA were detected. The gastric antrum was placed in formalin for pathological tissue analysis. The remaining tissues were stored in a refrigerator at -80°C.
2.3.3. Pharmacodynamics evaluation
The pH of gastric juice was measured by a high-precision desktop acidity meter. PA was detected according to the kit instructions. Gastric antrum tissue was embedded, sliced and stained by Servicebio Biotechnology Co., Ltd. (Hubei, Wuhan, China).
2.4. 16S rRNA sequencing of gastric mucosa
After the sample collection was completed, they were sent to Parsons Biotechnology Co., Ltd. (Shanghai, China) for 16S rRNA sequencing analysis. The analysis steps were summarized as follows: (1) the extraction of total DNA from microbiota, (2) polymerase chain reaction (PCR) amplification, (3) purification and recovery, (4) fluorescence quantification, (5) sequencing library preparation, and (6) sequencing on the machine.
The sequencing data were uploaded to the GeneCloud platform (www.genescloud.cn) by Parsono Biotechnology, and analyzed and mapped on the platform. One-way analysis of variance and T-test were used to analyze the differences in bacterial species, and p < 0.05 indicated a significant difference.
2.5. Metabolomics study of gastric tissue
2.5.1. Preparation of gastric tissue samples
100 mg of gastric tissue was put into an Ep tube and 0.5 mL of 75% methanol was added. After being put into the steel balls, the homogenate was homogenized using a high-throughput tissue grinding instrument. The mixture was vortexed for 1 min and 1.25 mL methyl tert-butyl ether (MTBE) was added. It was incubated in a metal bath (Speed: 1500, 25°C) for 30 min, ultrapure water (310 mL) was added. It was vortexed for 1 min, incubated in a metal bath for 10 mins, and then vortexed for 1 min. Then, the samples were equilibrated at room temperature for 5 mins, centrifuged at 4°C and 12,000 rpm for 15 mins, and 0.4 mL of the lower polar sample was taken and freeze-dried using a high-speed centrifugal freeze dryer. After lyophilization, 0.1 mL of 25% methanol was added to the sample, vortexed for 1 min, kept in an ultrasound for 15 mins, and 0.1 mL of the supernatant was taken for metabolomics analysis. A total of 0.01 mL was taken from each sample and vortexed as quality control (QC).
2.5.2. UHPLC-Q/TOF-MS analysis of gastric tissue
Liquid chromatography conditions were as follows: Waters ACQUITY UHPLC HSS T3 liquid chromatography column (2.1 mm × 100 mm, 1.8 μm) was used, the column temperature was 35°C, the mobile phase A was 1% formic acid water, B was 1% formic acid acetonitrile solution, the flow rate was 0.3 mL/min, and the injection volume was 2 μL. Elution gradient: 0 - 0.5 min, 1% B; 0.5 - 3.5min, 1% B - 53% B; 3.5 - 7.5 min, 53%B - 70% B; 7.5 - 10min, 70% B - 90% B; 10 - 13 min, 90% B; 13-14 min, 90% -1% B; 14 - 16 min, 1% B.
Mass spectrometry conditions were as follows: the ion source was electrospray ion source, and the acquisition mode was Sensitivity under MSE model. Scanning range: 50-1500 Da; collision voltage: 15.0-45.0 V; the Mass Range: 50-1500 Da; ion source temperature: 100°C; capillary voltage: 3.0 kV; cone voltage: 40 kV; desolvation temperature: 400°C; desolvation gas flow: 1000 L/h; MS/MS calibration solution: 200 pg/μL leucine enkephalin.
2.5.3. Data processing
The collected SDF files of all compounds were downloaded from the HMDB (http://www.hmdb.ca/) database, and the SDF files of all compounds were merged using Progenesis SDF Studio (Waters, USA) software as a database for identification. The raw format files were imported into Progenesis QI (Waters, USA) software for peak alignment, peak extraction and peak identification. Finally, the metabolites were identified according to the retention time, mass-to-charge ratio and secondary fragment matching with the database. The parameters were set as follows: m/z error: 10 ppm; retention time error: 0.05 min; relative mass error: 10 ppm. The peak table of the original data of the sample was derived from Progenesis QI (Waters, USA), and the online website (https://www.omicsolution.com/) was used to fill in the missing values and normalize the data. The processed data were imported into SIMCA (Umetrics, Sweden) software for supervised partial least squares discriminant analysis (PLS-DA) and orthogonal partial least squares discriminant analysis (OPLS-DA), and the accuracy of the model was tested by the corresponding order. The original data of control group and model group were analyzed by OPLS-DA contour analysis, VIP value was calculated, T-test test was performed with excel, and p value was calculated. Variables with VIP > 1 and p value < 0.05 were selected as differential variables and identified, and the results were used as potential pathological markers.
2.6. Metabolomics study of mitochondrial from gastric tissue
2.6.1. Extraction of mitochondrial samples
The mitochondria of gastric tissue were extracted using the tissue mitochondrial isolation kit (Shanghai, China) according to the kit instructions.
2.6.2. Preparation of mitochondrial samples
1 mL of pre-cold methanol was added to the mitochondria, shocked for 1 min, and placed on ice. The mitochondria were broken for 10 mins (broken for 5 s, suspended for 5 s) using an ultrasonic cell crusher, and centrifuged at 15000 g at 4°C for 15 mins. The supernatant was collected and lyophilized. Methanol (0.1 mL) was added to the freeze-dried sample for metabolomics analysis. 0.01 mL was extracted from each sample, and then vortexed as a QC sample for the detection of the stability of the instrument.
2.6.3. UHPLC-Q/TOF-MS analysis of mitochondrial sample
The liquid chromatography conditions were as follows: Waters ACQUITY UHPLC HSS T3 liquid chromatography column (2.1 mm × 100 mm, 1.8 μm) was used, the column temperature was 35°C, the mobile phase A was 1% formic acid water, B was 1% formic acid acetonitrile solution, the flow rate was 0.3 mL/min, and the injection volume was 2 μL. The elution gradient was as follows: 0 - 0.5 min, 99% A; 0.5 min - 3 min, 99% - 47% A; 3 min - 5 min, 47% - 10% A; 5 min - 12 min, 10% - 1% A; 12 min-16 min, 1% A; 16 min - 18 min, 1% - 99% A; 18 min - 20 min, 99% A.
The mass spectrometry conditions were the same as the above section.
2.6.4. Data processing
Mitochondrial metabolites were downloaded from the HMDB (https://hmdb.ca/) database as a database for identification.
2.7. Metabolite traceability analysis
Deep MetOrigin Analysis was performed at Metorigin (https://metorigin.met-bioinformatics.cn) with species set as Rat.
2.8. Construction of protein-protein interaction (PPI) network
Enzymes in co-metabolic pathways were collected in Metorigin. The enzyme information was imported into String (https://cn.string-db.org/), the species was set to Rat, the minimum required interaction score was set to medium confidence (0.400), and the hide disconnected nodes in the network. Import PPI network information into Cytoscape 3.10.1 for plotting.
2.9. Molecular docking
3D chemical structures of microbial metabolites were available for download in HMDB. The structure of the enzyme was downloaded from the PDB (http://www.rcsb.org/pdb/home/home.do) with the following information: purine-nucleoside phosphorylase (Pnp, PDB ID: 7zsl), glutathione reductase (Gsr, PDB ID: 3dk9), isocitrate dehydrogenase (Idh1, PDB ID: 4ja8), xanthine dehydrogenase/oxidase (Xdh, PDB ID: 2e1q), nicotinamide adenine dinucleotide kinase (Nadk, PDB ID: 7r4m), glutathione peroxidase (Gpx7, PDB ID: 3kij). Auto Blind Docking was performed at CB-Dock2 (https://cadd.labshare.cn/cb-dock2/php/index.php) and the docking score was recorded [28]. Docking scores less than -5 were considered to be combined pairs [29].
3. Results and Discussion
3.1. Pharmacodynamic evaluation
There was no obvious abnormality in the appearance of rats in the control group. The rats in the CAG group were apathetic, with yellow and dull hair, and thin body. The appearance of CAG could be improved by each experimental drug. The weight of rats in the model group was decreased significantly, and the other groups showed different degrees of improvement. The degree of improvement in each group was as follows in descending order: XJZ>XJZ-C>XJZ-P=Pos>XJZ-A (Figure 1a).
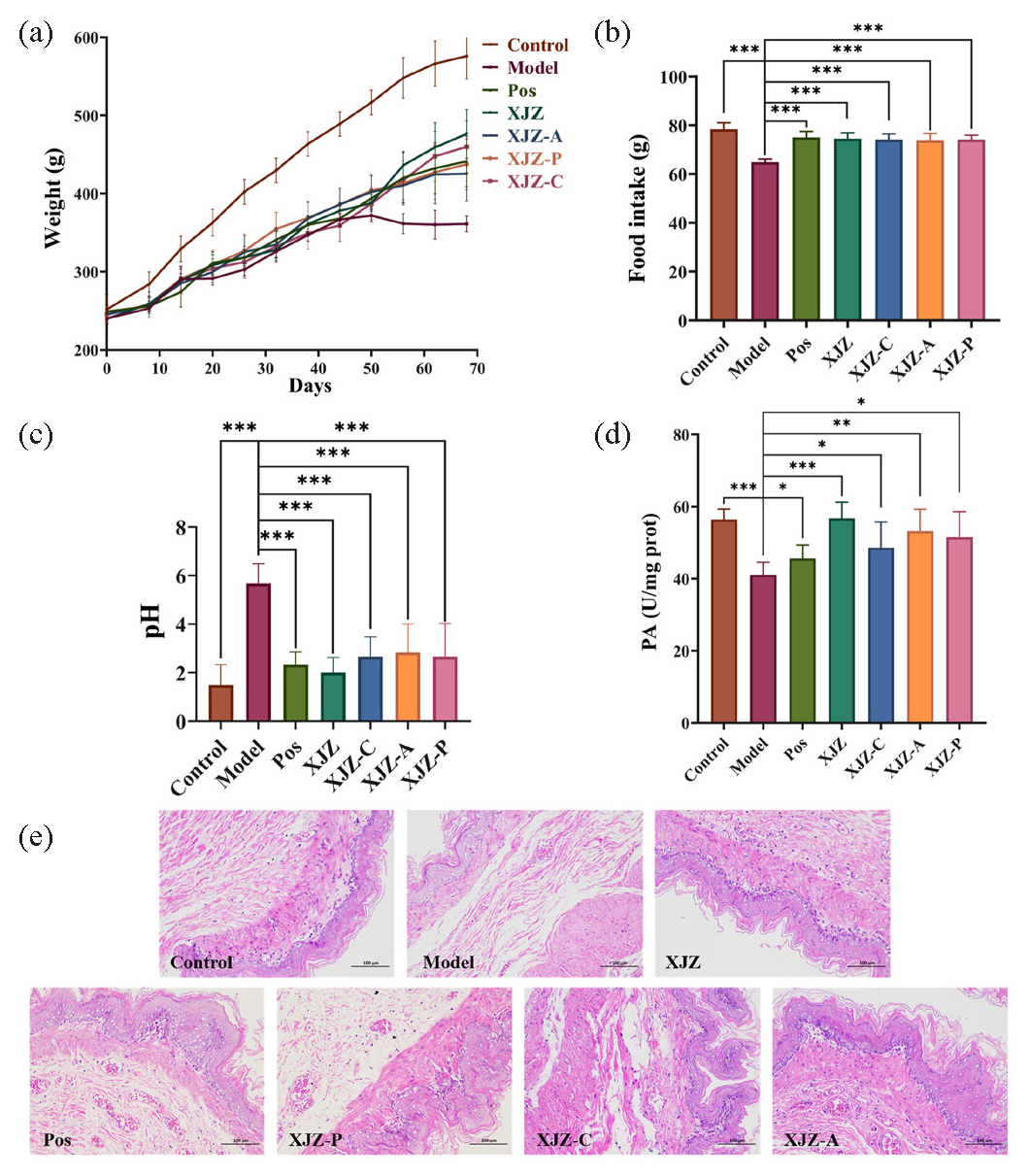
- Pharmacodynamic results. (a) Line graph of body weight change of rats. (b) Histogram of food intake of rats. (c) Histogram of pH of gastric juice of rats. (d) Histogram of PA. (e) Histopathological sections of stomach in rats stained with hematoxylin-eosin at 200X magnification. (Compared with model group, * p < 0.05, ** p < 0.01, *** p < 0.001, n=6).
Food intake of the model group was significantly lower than that of the control group. Food intake could be significantly increased by each experimental drug (Figure 1b).
The pH of the gastric juice was significantly higher in the model group compared to the control group. The pH of gastric juice could be significantly reduced by each experimental drug, with XJZ being the most effective (Figure 1c).
The PA was significantly reduced in the model group as compared with the control group. The PA improved with each experimental drug, with XJZ group being the best. The rest of the groups in descending order of action were as follows: XJZ-A, XJZ-P, XJZ-C and Pos (Figure 1d).
There was no abnormality in the gastric mucosa of rats in the control group. In the model group, the chromatin staining was light, the cell morphology and arrangement were irregular, the mucosal muscularis layer was atrophied, the submucosal layer was loose, and the mucosa was thinned. The pathological changes of the CAG could be improved with each experimental drug, among which the effect of XJZ was the most effective. The rest of the groups in descending order of action were as follows: XJZ-A, XJZ-C, Pos, and XJZ-P (Figure 1e).
3.2. XJZ and its subtracted formulas altered the microbiota of gastric mucosal
16S rRNA sequencing was performed to evaluate the effects of XJZ and its subtracted formulas on the gastric mucosal microbiota. In the Figure S2, the number of taxa has been shown in Figure S2(a). Venn diagram of ASV/OUT has been shown in Figure S2(b). The richness and diversity of the model rats were significantly decreased compared with control group (Figure 2a). While the alpha diversity could be modulated by XJZ, XJZ-A, and XJZ-C, but there was no significant modulation by XJZ-P. In the Beta diversity results, the control group was clearly separated from the model group; the colony structure was significantly regressed by XJZ, XJZ-A, and XJZ-C, and there was no significant regression by XJZ-P (Figure 2b). Heat maps of the phylum and genus level have been shown in Figure S2(c-d). The differential microbes in the model group compared to the control group included Actinobacteria, Fusobacteria, Tenericutes, Firmicutes, and Verrucomicrobia (Figure S2e). Actinobacteria, Verrucomicrobia, Fusobacteria, and Firmicutes could be significantly regulated by XJZ. The differential microbes in the model group compared to the control group included Cetobacterium, Corynebacterium, Jeotgalicoccus, Lactobacillus, Methylobacterium, Olsenella, Petrimonas, Rothia, and Streptococcus. Olsenella, Petrimonas, Rothia, and Streptococcus (Figure S2f). Corynebacterium, Cetobacterium, Jeotgalicoccus, Lactobacillus, Methylobacterium, Petrimonas, and Streptococcus could be significantly regulated by XJZ. The number of phylums regulated by XJZ-P, XJZ-A, and XJZ-C were 0, 1, and 3, respectively. The number of genera regulated by XJZ-P, XJZ-A, and XJZ-C were 3, 4, and 6, respectively.
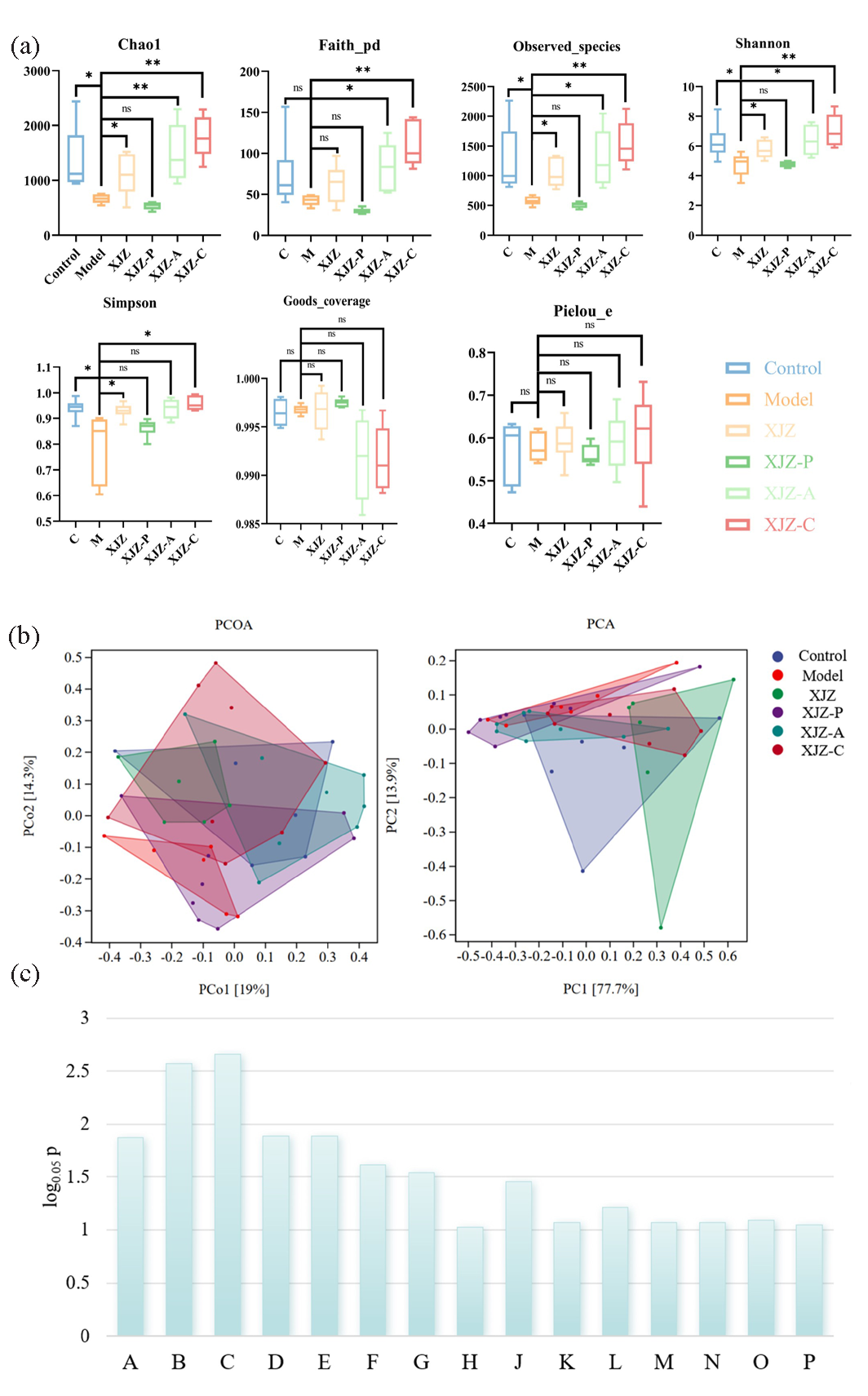
- Effect of XJZ and its subtracted formulas on gastric mucosal microbiota. (a) Alpha Diversity. (b) Beta diversity. (c) Analysis of microbial metabolic pathways in the gastric mucosa, metabolic pathways with log0.05 P values greater than 1 were considered statistically significant (A: Sulfur metabolism; B: Glycine, serine and threonine metabolism; C: Valine, leucine, and isoleucine biosynthesis; D: Secondary bile acid biosynthesis; E: Primary bile acid biosynthesis; F: Vitamin B6 metabolism; G: Nucleotide excision repair; H: Glycerophospholipid metabolism; J: Insulin signaling pathway; K: Pantothenate and CoA biosynthesis; L: Galactose metabolism; M: Pyrimidine metabolism; N: Glyoxylate and dicarboxylate metabolism; O: Peptidoglycan biosynthesis; P: D-Alanine metabolism) (Compared with model group, * p < 0.05, ** p < 0.01, *** p < 0.001, n=6).
The 16S rRNA gene sequences were used to predict metabolic pathways in the KEGG database, and a total of 15 differential metabolic pathways were predicted (Figure 2c). Each subtracted formula regulated the differential pathways weaker than XJZ. XJZ regulated all 15 differential metabolic pathways. No differential metabolic pathways were regulated by XJZ-P. After subtracting the “principal drug” from XJZ, microbial metabolic pathways were no longer regulated by XJZ-P. Nine of the 15 differential metabolic pathways were regulated by XJZ-A. After subtracting the “assistant drug” from XJZ, K, L, M, N, O, and P were no longer regulated by XJZ-A. Twelve of the 15 differential metabolic pathways could be regulated by XJZ-C. After subtracting the “complement drug” from XJZ, M, N, and O were no longer regulated by XJZ-C.
For CAG-induced gastric mucosal microbial disorders, the regulatory effects from high to low were XJZ, XJZ-C, XJZ-A, and XJZ-P.
3.3. Metabolomics analysis of rat gastric tissue
Metabolomic analysis of gastric tissues based on Waters Primer Synapt XS IMS-TOF technology was performed to investigate the effects of XJZ and its subtracted formulas on the metabolism of gastric tissues in CAG rats. LC-MS chromatograms of each group of gastric tissues in positive mode have been shown in Figure S3(a). The QC samples were all within 2-fold standard deviation (± 2SD) (Figure S3b). In the PLS-DA score plot, the control group was completely separated from the model group suggesting that CAG caused metabolic disturbances in gastric tissues (Figure 3a). In the permutation test results of PLS-DA, Q2 was smaller than R2 and the value of the intersection of Q2 with the Y-axis was negative, proving that the model was applicable (Figure 3b).
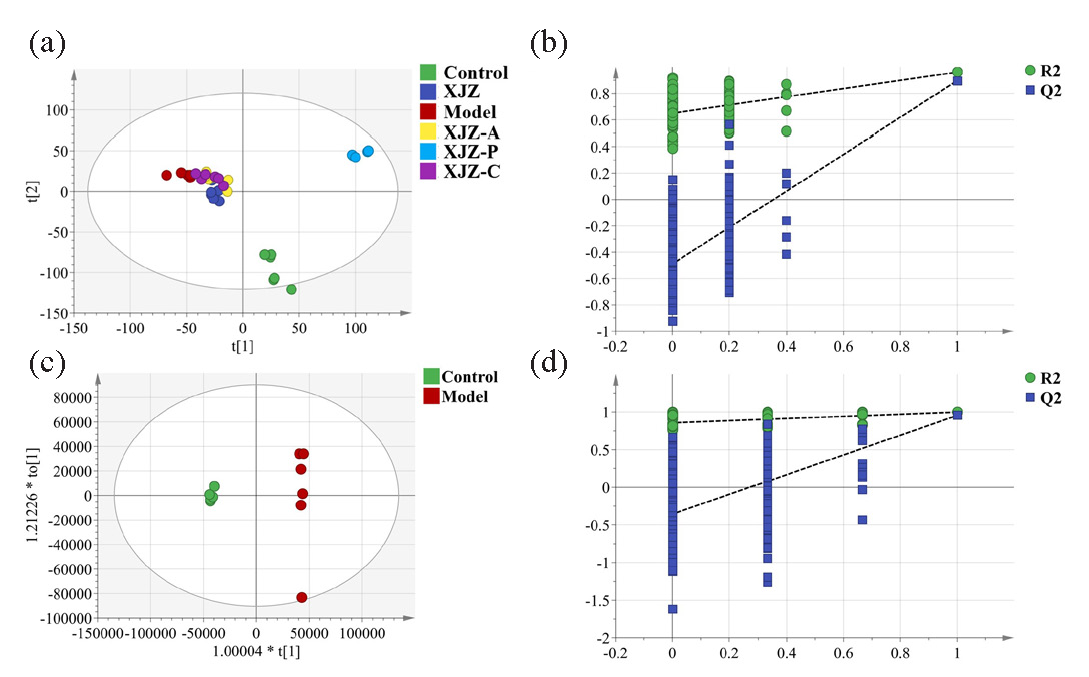
- Metabolomics analysis of gastric tissue. (a) PLS-DA of gastric tissue metabolomics. (b) Permutation test result of PLS-DA. (c) OPLS-DA of gastric tissue metabolomics (d) Permutation test result of OPLS-DA.
In the OPLS-DA score plot, the control group was completely separated from the model group (Figure 3c). The permutation test of OPLS-DA proved that the model was applicable (Figure 3d). Seventeen differential metabolites were identified, including cellobiose, chenodeoxycholic acid 3-sulfate, D-alanine, D-alanyl-d-alanine, glycoursodeoxycholic acid 3-sulfate, diaminopimelic acid, D-glutamic acid, deoxyglycocholate, phenylpyruvic acid, urobilinogen, L-homoserine, N2-succinyl-ornithine, hydroxypropionic acid, indoleacetic acid, taurodeoxycholic acid, N-methylnicotinamide, and glycocholic acid (Table S2). Nine compounds were significantly decreased and 8 compounds were significantly increased in the model group compared to the control group (Figure S3c). Seventeen differential metabolites were modulated by XJZ; glycoursodeoxycholic acid 3-sulfate and N-methylnicotinamide were modulated by all treatment groups with the same trend of modulation. The number of differential metabolites regulated by XJZ-P, XJZ-A, and XJZ-C were 3, 7, and 6, respectively. The results showed that the effects on the metabolic disorders of gastric tissue were XJZ, XJZ-A, XJZ-C, and XJZ-P in descending order of regulation.
3.4. Differential metabolite traceability and the STA-Sankey network
Six metabolites were host-associated and all six were “host-microbe” co-metabolites; 17 metabolites were microbe-associated, 11 of which were microbe-specific (Figure 4a and 4b, Table S3). 8 and 10 metabolite pathways belonged to microbiota and host-microbiota, respectively, of which 4 and 2 metabolic pathways were significantly (p < 0.05) associated with CAG, respectively (Figure 4c).
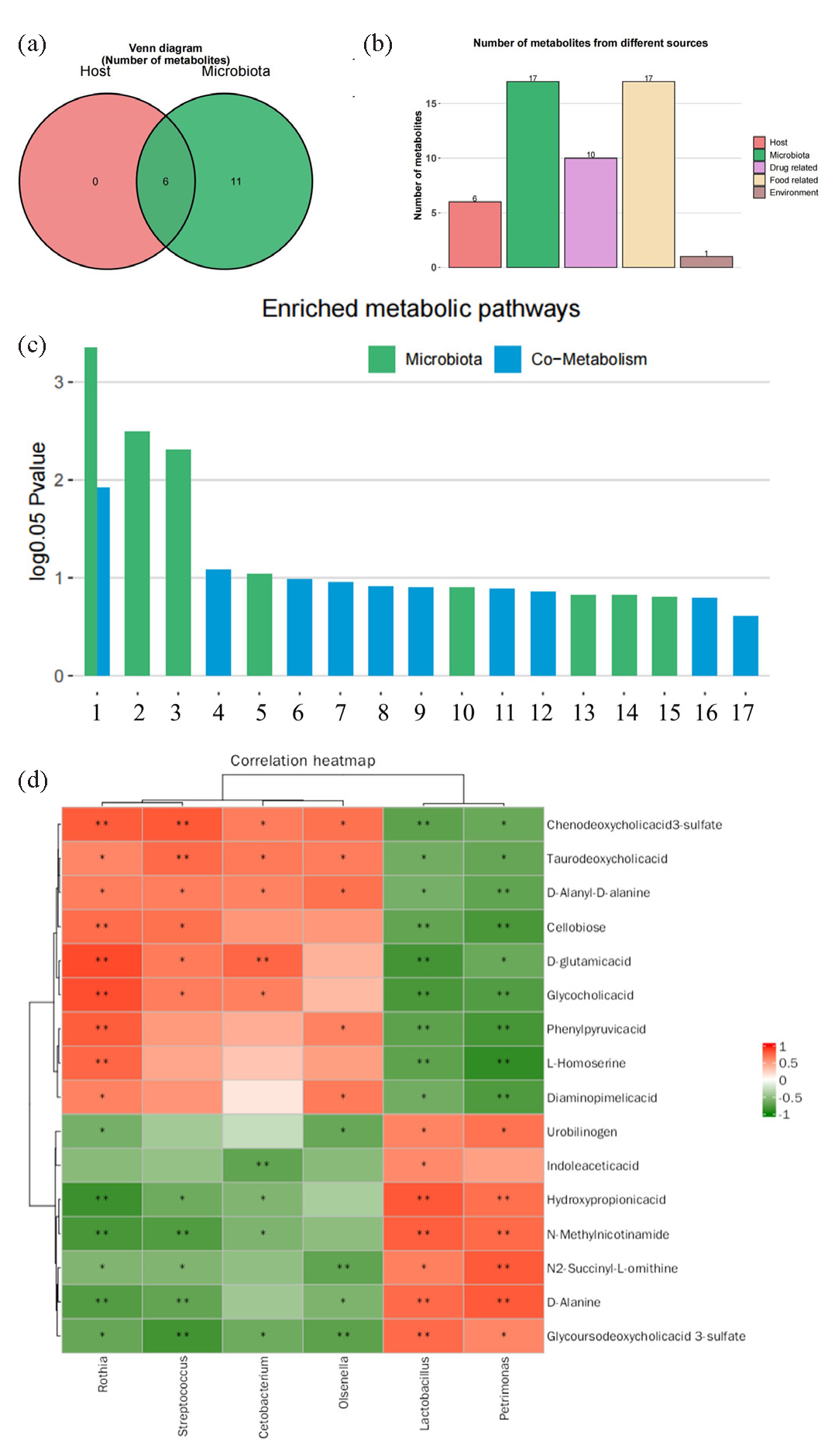
- MetOrigin analysis of differential metabolites in gastric tissues. (a) Venn diagram for traceability analysis. (b) Histogram of traceability analysis. (c) Functional analysis of differential metabolite traceability, metabolic pathways with log0.05 P values greater than 1 were considered statistically significant (1: D-Amino acid metabolism. 2: Peptidoglycan biosynthesis. 3: Lysine biosynthesis. 4: Beta−Alanine metabolism. 5: Sulfur metabolism. 6: Phenylalanine, tyrosine and tryptophan biosynthesis. 7: Propanoate metabolism. 8: Phenylalanine metabolism. 9: Carbon fixation pathways in prokaryotes. 10: Glycine, serine and threonine metabolism. 11: Primary bile acid biosynthesis. 12: Tryptophan metabolism. 13: Cysteine and methionine metabolism. 14: Teichoic acid biosynthesis. 15: Arginine and proline metabolism. 16: Pyrimidine metabolism. 17: Porphyrin metabolism). (d) Heatmap of microbial-metabolite correlation.
Significant metabolic pathways, including D-amino acid metabolism (involved metabolites: phenylpyruvicacid and D-glutamicacid) and Beta-alanine metabolism (involved metabolites: hydroxypropionicacid) were co-metabolised; D-amino acid metabolism (involved metabolites: D-alanyl-D-alanine, diaminopimelic acid, D-alanine), peptidoglycan biosynthesis (involved metabolites: D-alanyl-D-alanine; D-alanine), lysine biosynthesis (involved metabolites: diaminopimelic acid, L-homoserine), and sulfur metabolism (involved metabolite: L-homoserine) were microbial metabolism. Of interest, D-amino acid metabolism was the most prominent metabolic pathway in both co-metabolism and microbial metabolism, with a total of five involved metabolites, and was the most relevant pathway for CAG pathogenesis.
Cetobacterium was negatively correlated with indoleacetic acid and positively correlated with D-glutamic acid; Streptococcus was negatively correlated with D-alanine, N-methylnicotinamide, glycoursodeoxycholicacid 3-sulfate, and positively correlated with chenodeoxycholic acid 3-sulfate, taurodeoxycholic acid; Olsenella was negatively correlated with glycoursodeoxycholicacid 3-sulfate, N2-succinyl-ornithine and positively correlated with chenodeoxycholic acid 3-sulfate, taurodeoxycholic acid; Lactobacillus was positively correlated with hydroxypropionic acid and N-methylnicotinamide and negatively correlated with cellobiose and D-glutamic acid (Figure 4d). STA-Sankey network diagrams of D-Amino acid metabolism, beta-Alanine metabolism, Peptidoglycan biosynthesis, Lysine biosynthesis and Sulfur metabolism have been shown in Figure S4.
Based on the regulation of metabolites by each experimental drug, it was clear that all five metabolic pathways could be regulated by XJZ. 2 metabolites could be regulated by XJZ-P. D-amino acid metabolism, Beta−alanine metabolism, and peptidoglycan biosynthesis were not regulated by XJZ-P after subtracting “principal drug” from XJZ, suggesting that the “principal drug” plays a major role in the regulation of the above 3 metabolic pathways by XJZ. Three metabolic pathways could be regulated by XJZ-A. Lysine biosynthesis and sulfur metabolism were not regulated by XJZ-A after XJZ subtracted the “assistant drug”, suggesting that the “assistant drug” plays a major role in the regulation of the above 2 metabolic pathways by XJZ. Two metabolic pathways could be regulated by XJZ-C. Peptidoglycan biosynthesis, lysine biosynthesis, and sulfur metabolism were not regulated by XJZ-C after subtracting the “complement drug” from XJZ, suggesting that the “complement drug” played a major role in the regulation of the above 3 metabolic pathways by XJZ.
3.5. Metabolomics analysis of mitochondria
LC-MS chromatograms of each group of mitochondrial in positive mode have been shown in Figure S5(a). The QC samples were all within 2-fold standard deviation (± 2SD) (Figure S5b). The PLS-DA results for all the experimental groups have been shown in Figure 5(a), where the control and model groups were significantly separated. Permutation test has been shown in Figure 5(b), Q2 was less than R2 and Q2 intersects the Y-axis negatively, proving that the model was applicable.
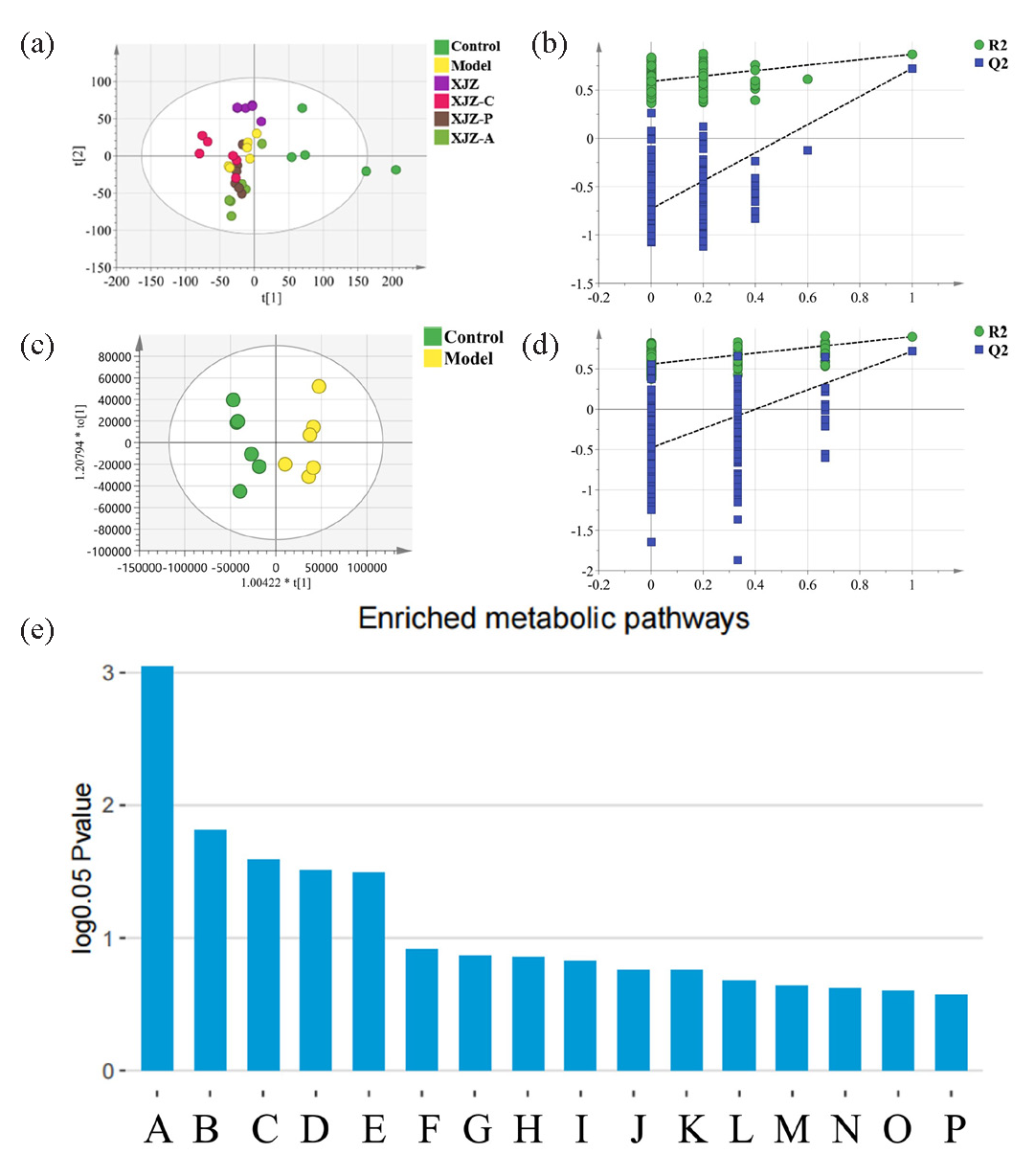
- Analysis of mitochondrial metabolomics. (a) PLS-DA of mitochondrial metabolomics. (b) Permutation test result of PLS-DA. (c) OPLS-DA of mitochondrial metabolomics. (d) Permutation test result of OPLS-DA. (e) Results of metabolic pathway enrichment analysis, metabolic pathways with log0.05 P values greater than 1 were considered statistically significant, (A: Purine metabolism; B: Glutathione metabolism; C: Biosynthesis of various other secondary metabolites; D: Nicotinate and nicotinamide metabolism; E: Aminoacyl-tRNA biosynthesis; F: Ether lipid metabolism; G: Biotin metabolism; H: Beta-Alanine metabolism; I: Pantothenate and CoA biosynthesis; J: Phenylalanine, tyrosine and tryptophan biosynthesis; K: Lysine biosynthesis; L: Phenylalanine metabolism; M: Glycerophospholipid metabolism; N: Lysine degradation; O: D-Amino acid metabolism; P: Pyrimidine metabolism).
In the OPLS-DA score plot, the control group was completely separated from the model group (Figure 5c). The permutation test of OPLS-DA proved that the model was applicable (Figure 5d). A total of 18 differential metabolites were identified, including glycerophosphocholine, deoxyguanosine, thymidine, L-carnitine, carnosine, guanine, pantothenic acid, glycylleucine, oxidized glutathione (GSSG), 1-methylnicotinamide, hypoxanthine, nicotinamide adenine dinucleotide phosphate (NADP), coenzyme Q10, lysine, phenylalanine, guanosine, adenosine, and Gamma-linolenyl carnitine (Table S4). Six compounds were decreased and 12 compounds were increased in the model group. The number of metabolites regulated by XJZ, XJZ-P, XJZ-A, and XJZ-C were 15, 7, 10, and 13, respectively (Figure S5c). For CAG-induced metabolic disorders, the modulatory effects were XJZ, XJZ-C, XJZ-A, and XJZ-P in descending order.
A total of 16 metabolic pathways were enriched, of which 5 metabolic pathways were significantly correlated with CAG (Figure 5e). Metabolic pathways included purine metabolism (involved metabolites: guanine, hypoxanthine, guanosine, adenosine and deoxyguanosine), glutathione metabolism (involved metabolites: GSSG and NADP), biosynthesis of various other secondary metabolites (involved metabolites: lysine and phenylalanine), nicotinate and nicotinamide metabolism (involved metabolites: 1-methylnicotinamide and NADP), and aminoacyl-tRNA biosynthesis (involved metabolites: lysine and phenylalanine). The most significant pathway was purine metabolism, suggesting that CAG was most affected by this pathway. All 5 pathways were regulated by XJZ, XJZ-A, and XJZ-C. Three metabolic pathways were regulated by XJZ-P, including nicotinate and nicotinamide metabolism, purine metabolism, and glutathione metabolism. Therefore, the mitochondrial metabolic pathway was most strongly regulated by “principal drug”.
3.6. “Microbiota-mitochondria” cross-talk
By combining 16S rRNA and metabolomics, changes in gastric mucosal microbial and gastric tissue metabolites were found, with all gastric tissue metabolites derived from microbes, as well as changes in mitochondrial metabolites. Many studies had found that microbes might influence mitochondrial function through metabolites [30,31]. Gastric tissue metabolites-mitochondrial metabolites correlation analysis revealed significant correlations between multiple microbial metabolites and multiple mitochondrial metabolites, suggesting that microbial metabolites could potentially influence mitochondrial metabolites (Figure 6a). We assumed that microbial metabolites could enter mitochondria to affect mitochondrial enzymes and mitochondrial function, and therefore collected enzyme proteins in the mitochondrial pathway for PPI and molecular docking analysis.
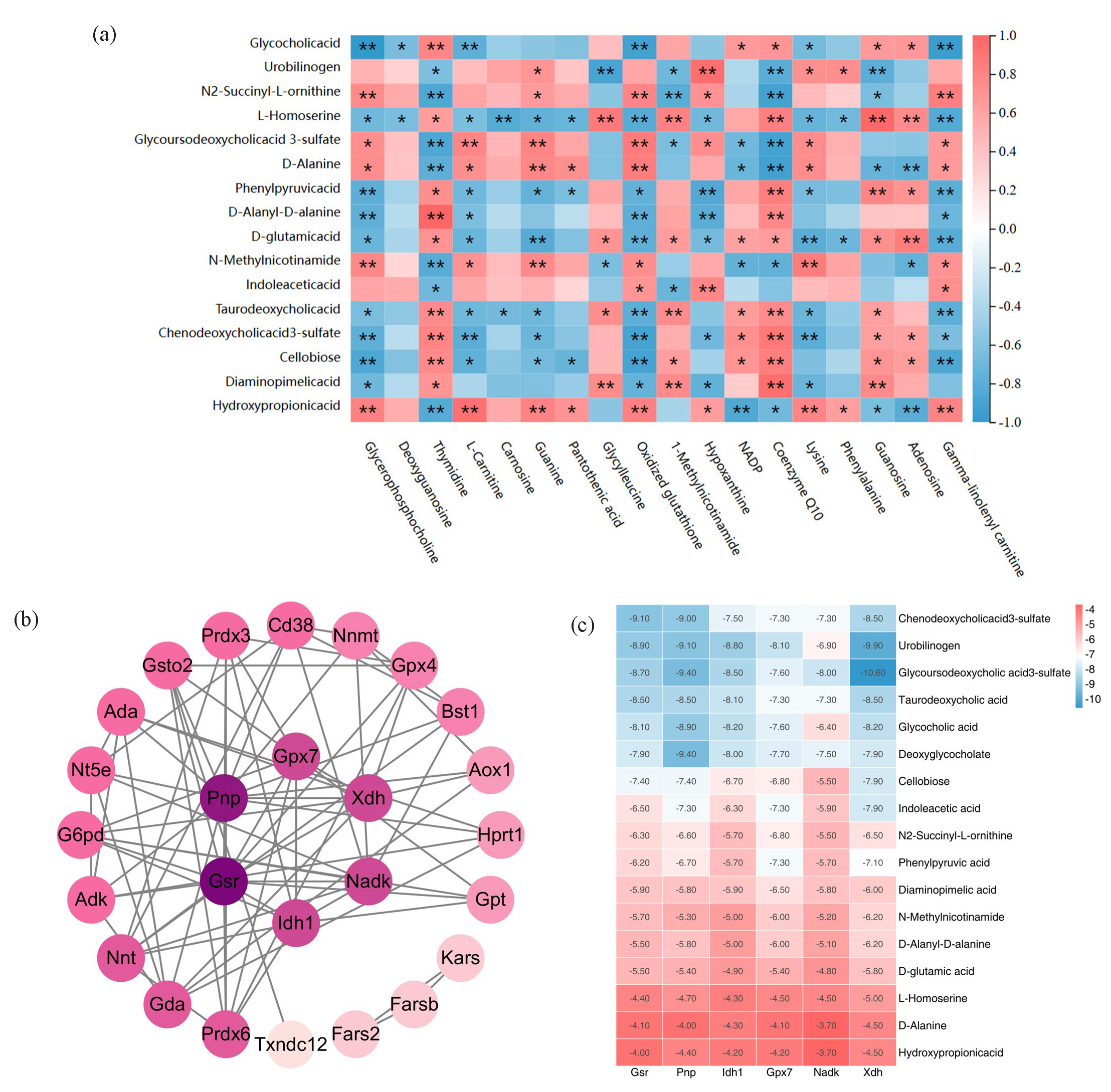
- ”Microbiota-mitochondria” cross-talk. (a) Correlation heat map of gastric tissue metabolites-mitochondrial metabolites. (b) PPI network diagram of mitochondria, the larger the degree, the darker the color. (c) Heat map of molecular docking scores.
The gene information of enzymes involved into mitochondrial metabolic pathway has been shown in Table S5. There were 26 nodes and 76 edges in the PPI network (Figure 6b). The top 6 of degree in the PPI network were selected as the key genes (degree ≥ 8) of “microbial-mitochondrial” cross-talk, including Gsr, Pnp, Idh1, Gpx7, Nadk, Xdh.
The molecular docking of microbial metabolites with key targets in mitochondria was shown in Figure 6(c), there were a total of 102 dockings, with 83 docking scores <-5 and 30 docking scores < -7.5. Mitochondrial targets were potential targets for microbial metabolites such as chenodeoxycholicacid3-sulfate, urobilinogen, and glycoursodeoxycholic acid 3-sulfate.
3.7. Regulation of CAG by components of the XJZ
The regulation of CAG by the subtracted formula was reduced after subtracting a certain part of the drug in XJZ. When a part of the drug was subtracted, the loss of a regulating effect could be regarded as this part of the drug having this regulating effect. The “principal drug,” “assistant drug,” and “complement drug” had different roles in the treatment of CAG by XJZ, but also co-regulate in many ways. For gastric microbiota, the number of genera individually regulated by “principal drug,” “assistant drug,” and “complement drug” were 2, 1, and 0, respectively. For gastric tissue metabolites, the number of gastric tissue metabolites individually regulated by “principal drug,” “assistant drug,” and “complement drug” were 4, 0, and 1, respectively. Eight gastric tissue metabolites were regulated by the “principal drug,” the “complement drug,” and the “assistant drug” together. For mitochondrial metabolites, the number of mitochondrial metabolites individually regulated by “principal drug,” “assistant drug,” and “complement drug” were 4, 1, and 0, respectively. Three mitochondrial metabolites were regulated by the “principal drug,” the “complement drug,” and the “assistant drug” together.
“Principal drug” could regulate Corynebacterium, Jeotgalicoccus, Lactobacillus, Streptococcus, and affect 14 microbial metabolites, such as deoxyglycocholate, phenylpyruvic acid, cellobiose, and taurodeoxycholic acid. These microbial metabolites could potentially affect Pnp, Xdh, Nadk, and influence purine metabolism and nicotinate and nicotinamide metabolism. It was worth noting that nicotinate and nicotinamide metabolism was only regulated by the “principal drug”.
“Assistant drug” could regulate Petrimonas, Streptococcus, Methylobacterium, Lactobacillus, and affect 10 microbial metabolites, such as deoxyglycocholate, cellobiose, and indoleacetic acid. These microbial metabolites could potentially affect Pnp, Xdh, Gsr, Idh1, Gpx7, and influence purine metabolism and glutathione metabolism.
“Complement drug” could regulate Streptococcus, Methylobacterium, and affect 11 microbial metabolites, such as cellobiose, chenodeoxycholic acid 3-sulfate, and N-methylnicotinamide. These microbial metabolites could potentially affect Gsr, Idh1, Gpx7, and influence glutathione metabolism.
Therefore the “principal drug” had the greatest role in the treatment of CAG with XJZ, the “assistant drug” and the “complement drug” alone had a lesser role, and both mainly played a role together with the “principal drug” (Figure 7).
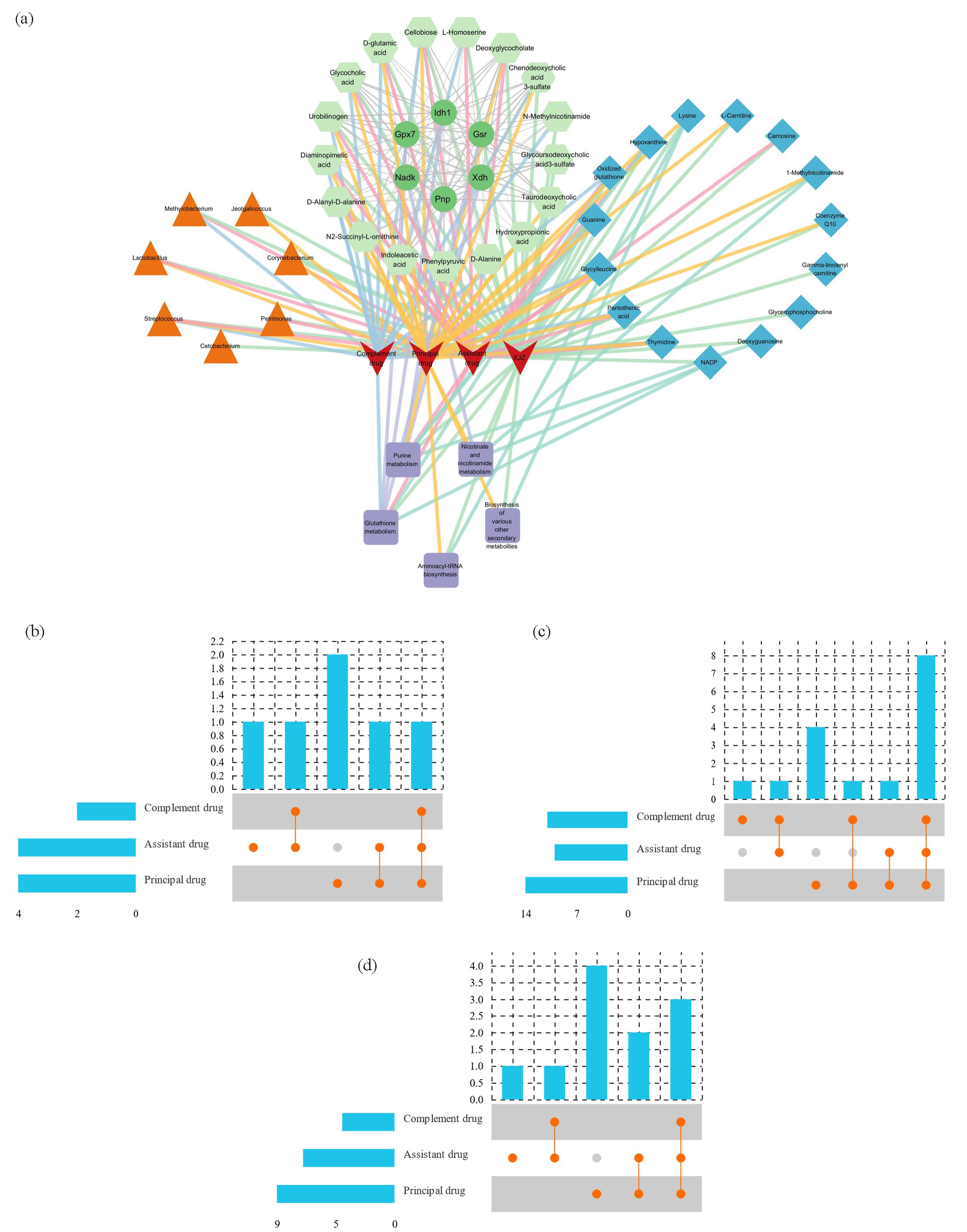
- (a) Network of regulation by XJZ, “principal drug”, “complement drug” and “assistant drug” (Red V: Drugs; Triangles: gastric mucosa microbes; Green hexagon: gastric tissue metabolites; Rhombus: mitochondrial metabolites; Purple rectangle: mitochondrial metabolite pathway; Green circle: potential target.). (b) Upset plots of genera-level microbes. (c) Upset plots of gastric tissue metabolites. (d) Upset plots of mitochondrial metabolites.
A multi-factorial approach was used in the study to establish a rat model of CAG, which were induced by combination administrations of sodium deoxycholate and ammonia coupled with starvation and fatigue. Deoxycholic acid is one of the most toxic components of reflux bile. 0.1% ammonia could mimic the damage to the gastric mucosa caused by high ammonia in the presence of Helicobacter pylori infection [32]. Several pharmacodynamic indicators showed that the rats in the model group had the disease characteristics of CAG, indicating that the model construction was successful.
Destruction of the gastric mucosa led to the death of acid-secreting wall cells and a decrease in gastric acid secretion, which could lead to overgrowth of certain bacteria [33], which was in accord with our work. The different microbes induced by CAG mainly involved Actinobacteria, Fusobacteria, Tenericutes, Firmicutes, and Verrucomicrobia at the phylum level, which were usually harmless to humans. Actinobacteria were widely distributed, produced abundant secondary metabolites, and played an important role in the energy cycle of ecosystems [34,35]. As an important microbial resource, Actinobacteria had important applications in the fields of medicine and biotechnology. Actinobacteria produced metabolites, such as alkaloids, terpenoids, esters, peptides, etc. [36,37], which were known to be anti-carcinogenic, anti-tumor, and anti-bacterial [38,39]. In the present study, reduced abundance of Actinobacteria in CAG rats reduced their effective metabolites, leading to exacerbation of CAG. Verrucomicrobia could utilize mucosal mucus as a source of nutrients [40], and its abundance was reduced in CAG rats due to the destruction of gastric mucosa by ammonia and deoxycholic acid. Systematic studies on Tenericutes were lacking, but their effects on CAG could not be ruled out.
The different microbes at the genera level in the control group compared to the model group included Cetobacterium, Corynebacterium, Jeotgalicoccus, Lactobacillus, Methylobacterium, Olsenella, Petrimonas, Rothia, and Streptococcus. Cetobacterium was a probiotic that produces vitamins, acetic acid, and butyric acid. CAG led to a decrease in Cetobacterium, which produced less acetic acid, leading to a block in participation in the tricarboxylic acid cycle [41]. The majority of Corynebacterium were pathogenic [42] and susceptible to infection in immunocompromised organisms, and their presence was increased in CAG. Lactobacillus was increased in CAG rats and it was a bacterium that produces bile acid hydrolase (BSH). Bound bile acids were increased in CAG rats [43] and BSH-producing bacteria were tolerant to bound bile acids [44]. Methylobacterium was pathogenic bacteria which was positively associated with liver disease [45] and could also cause kidney infections [46]. Olsenella was a probiotic reduced in CAG rats, and its elevation alleviated high-fat diet-induced obesity[47], hypercholesterolemia [48]. Streptococcus was a pathogenic bacterium whose cell wall complexes, secondary metabolites (hemolysin, autolysins, etc.) are harmful [49]. Rothia, Jeotgalicoccus and Petrimonas remain to be studied further.
To further explore the changes in gastric tissue metabolites, gastric tissue metabolomics analysis was performed. The results showed that CAG induced the metabolite disorders in gastric tissues, as well as the gastric mucosal flora. A total of 17 differential metabolites were identified, which were associated with the host and gastric mucosal flora.
Differential metabolite pathway analysis identified 5 metabolic pathways that were significantly associated with CAG and all of these pathways might be associated with microbes. D-amino acids regulated glucose metabolism by regulating insulin secretion [50,51]. In the present study, it was D-glutamate that was involved in the metabolism of D-amino acids, which for some time was thought to be metabolized by D-aspartate oxidase. However, a recent study showed that D-glutamate was also converted to 5-OXO-D-proline in mitochondria via a new D-glutamate cyclase [52]. 5-OXO-D-proline was associated with glutathione (GSH) in mitochondria [20], which was involved in mitochondrial redox reactions as a reducing substance in mitochondria. Peptidoglycan was an essential component of the cell wall of bacteria and was necessary for the maintenance of cell morphology, size, osmotic pressure stability, and cell survival [53,54]. In the host, peptidoglycan inhibited hexokinase (HK), which dissociated HK from mitochondria and therefore activated NLR family pyrin domain containing 3 (NLRP3) inflammasomes. NLRP3 inflammasomes regulated a number of mitochondrial functions including mitochondrial motility [55], mitochondrial division and growth [56], and mitochondrial autophagy [57]. Lysine biosynthesis could regulate the synthesis of lysine, which was important for regulating metabolic homeostasis in the body, enhancing the absorption of other proteins, promoting growth and development, and improving human dietary nutrition and animal nutrition [58]. Beta-alanine metabolism was a way of regulating Beta-alanine content. Beta-alanine was not involved in protein synthesis and was the only Beta-amino acid present in nature, involved in the composition of pantothenic acid, coenzyme A. In mice, Beta-alanine increased superoxide dismutase (SOD) and glutathione peroxidase (GSH-Px) activity in muscle, decreases oxidation products such as malondialdehyde (MDA), and improved muscular endurance [59]. Sulfur metabolism in microbes was associated with microbial resistance [60]. Sulfur existed in bacteria mainly in inorganic or organic form, inorganic substances included sulfate, thiosulfate and hydrogen sulfide (H2S), etc.; organic sulfur substances included various amino acids. H2S produced by bacteria was the substance that most directly affects the host, and H2S could stimulate the expression of complex IV (cytochrome c oxidase, CcO) in the mitochondria of trophoblast progenitor cells [61], and increased ATP production [62]. The above results suggested that gastric tissue metabolites were altered. Metabolites and metabolic pathways were associated with the gastric mucosal microbiota and potentially linked to mitochondrial metabolic alterations.
To investigate the changes in mitochondrial metabolites, mitochondrial metabolomics analysis was further performed, where total of 18 mitochondrial differential metabolites were identified. The differential metabolites were subjected to pathway analysis and five metabolic pathways were significantly correlated with CAG. In purine metabolism, purines were involved in the synthesis of a variety of biomolecules in the organism, such as nucleic acid synthesis, cell signals, cofactors, and co-transporters [63]. Purine metabolism was involved in the NLRP3 inflammatory pathway and the Cyclic adenosine monophosphate-protein kinase A (cAMP-PKA) pathway [64], which might account for its influence on CAG. Glutathione metabolism mainly involved the production of dihydronicotinamide-adenine dinucleotide phosphate (NADPH), glutathione, and GSSG, and was closely related to mitochondrial inflammation, oxidative stress, and apoptosis [65]. In biosynthesis of various other secondary metabolites, phenylalanine generates Cyclo (L-Trp-L-Phe). Cyclo (L-Trp-L-Phe) showed antimicrobial, antitumor, antiviral, and antioxidant effects, but its role in CAG is unknown. In this experiment, nicotinate and nicotinamide metabolism involved the synthesis of NADP and nicotinamide. NADP produced nicotinamide under the catalysis of ADP-ribosyl cyclase or cyclic ADP-ribose hydrol, and nicotinamide produced 1-methylnicotinamide under the catalysis of nicotinamide N-methyltra ferase catalyzed to produce 1-methylnicotinamide. The various types of niacinamide were collectively known as niacin, which is a vitamin. In CAG rats, the levels of 1-methylnicotinamide and NADP were significantly reduced, reflecting the fact that the overall level of niacin in their organisms was significantly decreased compared to normal rats. Lack of nicotinate could lead to a variety of diseases, such as enteritis, dermatitis, neuroinflammation [66]. Lack of nicotinate could be an important cause of CAG. In this study aminoacyl-tRNA biosynthesis involved the synthesis of L-phenylalanyl-tRNA and L-LySyI-tRNA, and the key to the pathway was aminoacyl-tRNA synthetase, which catalyzed the synthesis of aminoacyl-tRNA from amino acids and their corresponding tRNAs [67]. In CAG, mitochondrial phenylalanine and lysine were elevated, which suggested that their corresponding aminoacyl-tRNAs were blocked, which might lead to cessation of biological responses and mitochondrial dysfunction.
It had been shown that there existed a cross-talk between microbiota and mitochondria [68]. Mitochondria are essential organelles for ATP production and the main producers of reactive oxygen species. Bajpai et al. found that there was an overlap between abnormalities in intestinal flora and mitochondrial dysfunction, and that the intestinal flora might influence health and disease by regulating mitochondrial function [69]. Thiele et al. screened 2626 metabolites from 7439 reactions and found that 437 were from mitochondria, of which 325 overlapped with gut flora metabolites [31]. Microbiota can directly or indirectly affect mitochondria. Direct effects were manifested by microbial metabolites, such as SCFAs, H2S, NO, and secondary bile acids that could modulate mitochondrial function and affect host metabolism and health. Escherichia coli, Streptomyces and Pseudomonas aeruginosa in the intestine secreted xanthine oxidase, which converts purines in the intestine to uric acid [70]. Uric acid was reducibility and had the function of scavenging many reactive oxygen species such as oxygen radicals and peroxynitrite produced by mitochondria [71]. CAG caused thinning of gastric mucosa, reduction of barrier function, and entry of a large amount of lipopolysaccharide (LPS) produced by bacteria into gastric tissue cells. LPS could induce NF-κB-mediated inflammation [72] and increase intracellular ROS and activate the ROS/p38/MAPK signaling pathway, which affected apoptosis [73]. SCFAs produced by microbiota affect mitochondrial function, for example, the lack of butyrate led to a significant reduction in nicotinamide adenine dinucleotide (NAD), resulting in autophagy in germ-free mouse colon cells [30]. It had also been shown that microbes regulated mitochondrial function by indirectly inducing genes of SIRT1, FIAF, and FXR [74].
To further explore the effects of microbial metabolites on mitochondrial enzymes, molecular docking was used. Gsr was an oxidoreductase enzyme that was an important component of the human redox system. With NADPH as a cofactor, Gsr catalyzed the formation of reduced GSH from GSSG, which is beneficial to the body’s antioxidant activity [75]. Pnp was a key enzyme in the purine remediation pathway, catalyzing the phosphorylation of nucleosides, such as inosine and guanosine and participating in the degradation of ATP [76]. Idh1 was located in the mitochondrial matrix and converted isocitrate to α-ketoglutarate (α-KG) and NADP to NADPH. α-KG was a cofactor for many dehydrogenases and was involved in the regulation of various key biological processes, including nucleic acid repair, hypoxia response and chromatin modification and fatty acid metabolism. α-KG could regulate various key biological processes, including nucleic acid repair, hypoxia response, chromatin modification and fatty acid metabolism. During the conversion of isocitrate to α-KG, Idh1 catalyzed the generation of NADPH, and NADPH and reduced nicotinamide adenine dinucleotide (NADH) were reductants for lipid biosynthesis and antioxidants that protect cells from oxidative stress and radiation damage [77]. Gpx was an important oxidoreductase containing eight family members that played important roles in scavenging peroxides and maintaining the functional integration of cell membranes [78]. High expression of Gpx7 could alleviate cellular damage caused by ROS, and Gpx7 also reduced IL-1β-induced cellular inflammation, apoptosis, and extracellular matrix degradation through the regulation of iron death [79]. Nadk was the rate-limiting enzyme that phosphorylated NAD to NADP and is highly conserved throughout biological evolution. NADP was important in cellular redox homeostasis [80]. The main role of Xdh was to act as a catalyst for the conversion of hypoxanthine to xanthine, and ultimately to uric acid [81]. Multiple microbial metabolites could dock with the six enzyme proteins mentioned above, suggesting that there was a potential cross-talk between microbiota and mitochondria.
XJZ enhanced the antioxidant and anti-inflammatory capacity of piglet liver through Nrf2/NF-κB pathway [82]. XJZ reduced mucosal damage, increased ZO-1 and occludin expression, decreased epithelial cell apoptosis, decreased the expression of autophagy-related proteins LC3 and beclin1, increased the expression of p62, activated the PI3K/AKT/mTOR/ULK1 (ser757) signaling pathway, inhibited AMPK/ULK1 (ser317) signaling pathway, antagonized the imbalance of redox homeostasis, and protected the gastric mucosa [83]. In this study, it was found that the effect of XJZ against CAG was the result of the participation of all parts. The “principal drug” had the strongest effect, and the “assistant drug” and the “complement drug” were weaker and more auxiliary to the “principal drug” compared to compared to the “principal drug”. Although the effect of the “principal drug” was the strongest, the effect of XJZ was reduced without the assistance of the “assistant drug” and the “complement drug”. The treatment of TCM compound was a holistic action of all the parts, with possible synergies between the parts [84].
Additionally, in other studies of CAG, metabolomics analysis had been conducted using samples, such as serum and urine, and many CAG biomarkers had been identified. Interestingly and similarly, differences in energy metabolism, such as glycerophospholipid metabolism, citrate cycle, and alanine, aspartate, and glutamate metabolism, were also observed in serum and urine between normal and CAG rats in these studies [85,86]. Serum samples provided a more practical and simpler choice for disease monitoring, which was very important in clinical practice. Compared with serum or urine samples, the gastric tissue can offer a unique perspective on localized metabolic information. The metabolic profiles of gastric tissue could offer a direct description of its pathological process during the onset and progression of CAG, and facilitate the understanding of the underlying mechanism of CAG. Our current research focused on gastric tissue to gain a deeper understanding of the metabolic links between the gastric tissues and microbes, as well as the cross-talk between gastric mucosal microbes and mitochondria. These findings might provide new ideas for the mechanism of XJZ and its subtracted formulas in the treatment of CAG. The connection between gastric tissue and serum would be performed to provide a more comprehensive and deeper lights into the efficacy of XJZ against CAG, as well as its compatibility.
In future experiments, molecular biology experiments will be performed to validate the results of molecular docking and Metorigin. The mechanism of cross-talk between mitochondria and microbes needs to be further investigated. In addition, we need to go deeper into the function and efficacy of the compounds in XJZ.
4. Conclusions
In this study, 16S rRNA sequencing, metabolomics, metabolite traceability, and molecular docking techniques were integrated to demonstrate that there was a cross-talk between gastric mucosal microbes and mitochondria. XJZ and its subtracted formulas improved CAG-induced mitochondrial dysfunction, which may be mediated by microbial metabolites (Graphical abstract). In the treatment of CAG with XJZ, the role of the “principal drug” was the greatest, and the “assistant drug” and “complement drug” could assist the “principal drug” to work.
CRediT authorship contribution statement
Wentian Lu: Conceptualization, Data curation, Investigation, Methodology, Visualization and Writing - original draft; Hui Zhang: Conceptualization, Data curation, and Investigation; Junjie Guo: Writing - review & editing; Xuemei Qin: Writing - review & editing; Yuetao Liu: Conceptualization, Funding acquisition, Investigation, Project administration and Writing - review & editing. All authors agree to be accountable for all aspects of work ensuring integrity and accuracy.
Declaration of competing interest
The authors declare that they have no known competing financial interests or personal relationships that could have appeared to influence the work reported in this paper.
Declaration of Generative AI and AI-assisted technologies in the writing process
The authors confirm that there was no use of artificial intelligence (AI)-assisted technology for assisting in the writing or editing of the manuscript and no images were manipulated using AI.
Acknowledgment
This work was supported by the National Natural Science Foundation of China (82073988).
Supplementary data
Supplementary material to this article can be found online at https://dx.doi.org/10.25259/AJC_65_2024.
References
- Bile reflux is an independent risk factor for precancerous gastric lesions and gastric cancer: An observational cross‐sectional study. Journal of Digestive Diseases. 2021;22:282-290. https://doi.org/10.1111/1751-2980.12986
- [Google Scholar]
- Distinct metaplastic and inflammatory phenotypes in autoimmune and adenocarcinoma‐associated chronic atrophic gastritis. United European Gastroenterology Journal. 2017;5:37-44. https://doi.org/10.1177/2050640616644142
- [Google Scholar]
- Chronic atrophic gastritis: A review. Journal of Environmental Pathology, Toxicology and Oncology. 2018;37:241-259. https://doi.org/10.1615/JEnvironPatholToxicolOncol.2018026839
- [Google Scholar]
- Oral arsenic plus retinoic acid versus intravenous arsenic plus retinoic acid for non-high-risk acute promyelocytic leukaemia: A non-inferiority, randomised phase 3 trial. The Lancet Oncology. 2018;19:871-9. https://doi.org/10.1016/S1470-2045(18)30295-X
- [Google Scholar]
- Pharmacopoeia of People’s Republic of China. Beijing: China Medical. Science Press; 2020.
- Bile acid metabolism involved into the therapeutic action of Xiaojianzhong Tang via gut microbiota to treat chronic atrophic gastritis in rats. Phytomedicine. 2023;109:154557. https://doi.org/10.1016/j.phymed.2022.154557
- [Google Scholar]
- Unidentified curved bacilli in the stomach of patients with gastritis and peptic ulceration. Lancet. 1984;1:1311-5. https://doi.org/10.1016/s0140-6736(84)91816-6
- [Google Scholar]
- Systematic review: Gastric microbiota in health and disease. Alimentary Pharmacology & Therapeutics. 2020;51:582-602. https://doi.org/10.1111/apt.15650
- [Google Scholar]
- Molecular analysis of the bacterial microbiota in the human stomach. Proceedings of the National Academy of Sciences. 2006;103:732-7. https://doi.org/10.1073/pnas.0506655103
- [Google Scholar]
- Mitochondria in health, disease, and aging. Physiological Reviews. 2023;103:2349-2422. https://doi.org/10.1152/physrev.00058.2021
- [Google Scholar]
- Mitochondrial biogenesis in neurodegeneration. Journal of Neuroscience Research. 2017;95:2025-9. https://doi.org/10.1002/jnr.24042
- [Google Scholar]
- An overview: The diversified role of mitochondria in cancer metabolism. International Journal of Biological Sciences [electronic Resource]. 2023;19:897-915. https://doi.org/10.7150/ijbs.81609
- [Google Scholar]
- Atrophic gastritis: deficient complex I of the respiratory chain in the mitochondria of corpus mucosal cells. Journal of Gastroenterology. 2008;43:780-8. https://doi.org/10.1007/s00535-008-2231-4
- [Google Scholar]
- Network pharmacology research of Astragali Radix in treating chronic atrophic gastritis rats based on mitochondrial metabonomics. Journal of Chromatography B. 2020;1145:122109. https://doi.org/10.1016/j.jchromb.2020.122109
- [Google Scholar]
- Short-chain fatty acids in control of body weight and insulin sensitivity. Nature Reviews Endocrinology. 2015;11:577-591. https://doi.org/10.1038/nrendo.2015.128
- [Google Scholar]
- The SCFA receptor GPR43 and energy metabolism. Front Endocrinol (Lausanne). 2014;5:85. https://doi.org/10.3389/fendo.2014.00085
- [Google Scholar]
- Study on the mechanism of action of Xiaojianzhong Tang in treating chronic atrophic gastritis rats based on the regulation of gastrointestinal microbiota. Thesis for Master’ s degree. 2023 https://link.cnki.net/doi/10.27284/d.cnki.gsxiu.2023.000152
- [Google Scholar]
- Digestion characteristics of jujube polysaccharide and its regulatory effect on intestinal microbiota and metabolites during in vitro fermentation. LWT. 2024;210:116869. https://doi.org/10.1016/j.lwt.2024.116869
- [Google Scholar]
- Zingiber officinale: A systematic review of botany, phytochemistry and pharmacology of gut microbiota-related gastrointestinal benefits. The American Journal of Chinese Medicine (Gard City N Y). 2022;50:1007-1042. https://doi.org/10.1142/S0192415X22500410
- [Google Scholar]
- Integrating metabolomics, 16S rRNA sequencing, network pharmacology, and metorigin to explore the mechanism of Cinnamomi Cortex in treating chronic atrophic gastritis rats. Phytomedicine. 2023;121:155084. https://doi.org/10.1016/j.phymed.2023.155084
- [Google Scholar]
- Saccharum Granorum ameliorated mitochondrial dysfunction in chronic atrophic gastritis rats using organelle-specific metabolomics and network pharmacology approaches. Food Research International. 2020;136:109503. https://doi.org/10.1016/j.foodres.2020.109503
- [Google Scholar]
- Glycyrrhiza uralensis Fisch. alleviates dextran sulfate sodium-induced colitis in mice through inhibiting of NF-κB signaling pathways and modulating intestinal microbiota. Journal of Ethnopharmacology. 2022;298:115640. https://doi.org/10.1016/j.jep.2022.115640
- [Google Scholar]
- Aqueous extract of Paeoniae Radix Alba (Paeonia lactiflora Pall.) ameliorates DSS-induced colitis in mice by tunning the intestinal physical barrier, immune responses, and microbiota. Journal of Ethnopharmacology. 2022;294:115365. https://doi.org/10.1016/j.jep.2022.115365
- [Google Scholar]
- Metabolomics biotechnology, applications, and future trends: A systematic review. RSC Advances. 2019;9:37245-37257. https://doi.org/10.1039/C9RA06697G
- [Google Scholar]
- MetOrigin: Discriminating the origins of microbial metabolites for integrative analysis of the gut microbiome and metabolome. iMeta. 2022;1:e10. https://doi.org/10.1002/imt2.10
- [Google Scholar]
- Enhancing coverage of annotated compounds in traditional Chinese medicine formulas: Integrating MSE and Fast-DDA molecular network with AntDAS—Case study of Xiao Jian Zhong Tang. Journal of Chromatography A. 2024;1738:465498. https://doi.org/10.1016/j.chroma.2024.465498.
- [Google Scholar]
- Dose translation from animal to human studies revisited. The FASEB Journal. 2008;22:659-661. https://doi.org/10.1096/fj.07-9574LSF
- [Google Scholar]
- CB-Dock2: improved protein–ligand blind docking by integrating cavity detection, docking and homologous template fitting. Nucleic Acids Research. 2022;50:W159-W164. https://doi.org/10.1093/nar/gkac394
- [Google Scholar]
- Combining machine learning systems and multiple docking simulation packages to improve docking prediction reliability for network pharmacology. PloS One. 2013;8:e83922. https://doi.org/10.1371/journal.pone.0083922
- [Google Scholar]
- The microbiome and butyrate regulate energy metabolism and autophagy in the mammalian colon. Cell Metabolism. 2011;13:517-526. https://doi.org/10.1016/j.cmet.2011.02.018
- [Google Scholar]
- A community-driven global reconstruction of human metabolism. Nature Biotechnology. 2013;31:419-425. https://doi.org/10.1038/nbt.2488
- [Google Scholar]
- Helicobacter pylori-associated ammonia production enhances neutrophil-dependent gastric mucosal cell injury. American Journal of Physiology-Gastrointestinal and Liver Physiology. 1992;263:G719-G725. https://doi.org/10.1152/ajpgi.1992.263.5.G719
- [Google Scholar]
- Chronic atrophic gastritis: Natural history, diagnosis and therapeutic management. A position paper by the Italian Society of Hospital Gastroenterologists and Digestive Endoscopists [AIGO], the Italian Society of Digestive Endoscopy [SIED], the Italian Society of Gastroenterology [SIGE], and the Italian Society of Internal Medicine [SIMI] Digestive and Liver Disease. 2019;51 1621–1632. https://doi.org/10.1016/j.dld.2019.09.016
- [Google Scholar]
- Selective isolation, antimicrobial screening and phylogenetic diversity of marine actinomycetes derived from the coast of Bejaia City (Algeria), a polluted and microbiologically unexplored environment. Journal of Applied Microbiology. 2022;132:2870-2882. https://doi.org/10.1111/jam.15415
- [Google Scholar]
- Comparative transcriptomic analysis of two Saccharopolyspora spinosa strains reveals the relationships between primary metabolism and spinosad production. Scientific Reports. 2021;11:14779. https://doi.org/10.1038/s41598-021-94251-z
- [Google Scholar]
- Marinoterpins A–C: Rare linear merosesterterpenoids from marine-derived actinomycete bacteria of the family Streptomycetaceae. Journal of Organic Chemistry. 2021;86:11140-48. https://doi.org/10.1021/acs.joc.1c00262
- [Google Scholar]
- Secondary metabolites and biodiversity of actinomycetes. Journal of Genetic Engineering and Biotechnology. 2021;19:72. https://doi.org/10.1186/s43141-021-00156-9
- [Google Scholar]
- Isolation of actinomycetes with cellulolytic and antimicrobial activities from soils collected from an urban green space in the Philippines. International Journal of Microbiology. 2021;2021:1-14. https://doi.org/10.1155/2021/6699430
- [Google Scholar]
- Evaluation of the anticarcinogenic potential of the endophyte, Streptomyces sp. LRE541 isolated from Lilium davidii var. unicolor (Hoog) Cotton. Microbial Cell Factories [electronic Resource]. 2021;20:217. https://doi.org/10.1186/s12934-021-01706-z
- [Google Scholar]
- Microbes inside—from diversity to function: the case of Akkermansia. The ISME Journal. 2012;6:1449-1458. https://doi.org/10.1038/ismej.2012.6
- [Google Scholar]
- Modulation of short-chain fatty acids as potential therapy method for type 2 diabetes mellitus. Canadian Journal of Infectious Diseases and Medical Microbiology. 2021;2021:1-13. https://doi.org/10.1155/2021/6632266
- [Google Scholar]
- Dysbiosis and Staphylococcus aureus colonization drives inflammation in atopic dermatitis. Immunity. 2015;42:756-766. https://doi.org/10.1016/j.immuni.2015.03.014
- [Google Scholar]
- Autoregulatory control of mitochondrial glutathione homeostasis. Science. 2023;(1979) 382:820-28. https://doi.org/10.1126/science.adf4154
- [Google Scholar]
- The lactobacillus bile salt hydrolase repertoire reveals niche-specific adaptation. mSphere. 2018;3:e00140-18. https://doi.org/10.1128/mSphere.00140-18
- [Google Scholar]
- Gut microbiome dysbiosis in patients with hepatitis B virus-related hepatocellular carcinoma after extended hepatectomy liver failure. Annals of Translational Medicine. 2022;10:549. https://doi.org/10.21037/atm-22-1958
- [Google Scholar]
- Methylobacterium infection-induced peritonitis in a patient with renal insufficiency: A case report and literature review. Int. Journal of Clinical Pharmacology and Therapeutics. 2024;62:96-100. https://doi.org/10.5414/CP204483
- [Google Scholar]
- Probiotic-fermented tomato alleviates high-fat diet-induced obesity in mice: Insights from microbiome and metabolomics. Food Chemistry. 2024;436:137719. https://doi.org/10.1016/j.foodchem.2023.137719
- [Google Scholar]
- Lactiplantibacillus plantarum ATCC8014 alleviates postmenopausal hypercholesterolemia in mice by remodeling intestinal microbiota to increase secondary bile acid excretion. Journal of Agricultural and Food Chemistry. 2024;72:6236-6249. https://doi.org/10.1021/acs.jafc.3c08232
- [Google Scholar]
- Pathogenesis, epidemiology and control of Group A Streptococcus infection. Nature Reviews Microbiology. 2023;21:431-447. https://doi.org/10.1038/s41579-023-00865-7
- [Google Scholar]
- Immunohistochemical localization of d-alanine to β-cells in rat pancreas. Biochemical and Biophysical Research Communications. 2007;355:872-6. https://doi.org/10.1016/j.bbrc.2007.02.056
- [Google Scholar]
- Chronic d-serine supplementation impairs insulin secretion. Molecular Metabolism. 2018;16:191-202. https://doi.org/10.1016/j.molmet.2018.07.002
- [Google Scholar]
- D-Glutamate is metabolized in the heart mitochondria. Scientific Reports. 2017;7:43911. https://doi.org/10.1038/srep43911
- [Google Scholar]
- Regulation of peptidoglycan synthesis and remodelling. Nature Reviews Microbiology. 2020;18:446-460. https://doi.org/10.1038/s41579-020-0366-3
- [Google Scholar]
- From the regulation of peptidoglycan synthesis to bacterial growth and morphology. Nature Reviews Microbiology. 2012;10:123-136. https://doi.org/10.1038/nrmicro2677
- [Google Scholar]
- Microtubule-driven spatial arrangement of mitochondria promotes activation of the NLRP3 inflammasome. Nature Immunology. 2013;14:454-460. https://doi.org/10.1038/ni.2550
- [Google Scholar]
- Defective mitochondrial fission augments NLRP3 inflammasome activation. Scientific Reports. 2015;5:15489. https://doi.org/10.1038/srep15489
- [Google Scholar]
- NF-κB Restricts inflammasome activation via elimination of damaged mitochondria. Cell. 2016;164:896-910. https://doi.org/10.1016/j.cell.2015.12.057
- [Google Scholar]
- Review of lysine metabolism with a focus on humans. Journal of Nutrition. 2020;150:2548S-2555S. https://doi.org/10.1093/jn/nxaa224
- [Google Scholar]
- Influence of different histidine sources and zinc supplementation of broiler diets on dipeptide content and antioxidant status of blood and meat. British Poultry Science. 2013;54:454-465. https://doi.org/10.1080/00071668.2013.793295
- [Google Scholar]
- Hydrogen sulfide, reactive sulfur species and coping with reactive oxygen species. Free Radical Biology & Medicine. 2019;140:74-83. https://doi.org/10.1016/j.freeradbiomed.2019.01.020
- [Google Scholar]
- AP39, a modulator of mitochondrial bioenergetics, reduces antiangiogenic response and oxidative stress in hypoxia-exposed trophoblasts. American Journal of Pathology. 2019;189:104-114. https://doi.org/10.1016/j.ajpath.2018.09.007
- [Google Scholar]
- S- Sulfhydration of ATP synthase by hydrogen sulfide stimulates mitochondrial bioenergetics. Pharmacological Research : the Official Journal of the Italian Pharmacological Society. 2016;113:116-124. https://doi.org/10.1016/j.phrs.2016.08.023
- [Google Scholar]
- Mass spectrometric analysis of purine de novo biosynthesis intermediates. PloS One. 2018;13:e0208947. https://doi.org/10.1371/journal.pone.0208947
- [Google Scholar]
- New perspectives in signaling mediated by receptors coupled to stimulatory G protein: the emerging significance of cAMP eï„ux and extracellular cAMP-adenosine pathway. Frontiers in Pharmacology. 2015;6:58. https://doi.org/10.3389/fphar.2015.00058
- [Google Scholar]
- Glutathione in brain disorders and aging. Molecules. 2022;27:324. https://doi.org/10.3390/molecules27010324
- [Google Scholar]
- Niacin – A scoping review for nordic nutrition recommendations 2023. Food & Nutrition Research. 2023;67 https://doi.org/10.29219/fnr.v67.10299
- [Google Scholar]
- Aminoacyl-tRNA synthetases. RNA (New York, N.Y.). 2020;26:910-936. https://doi.org/10.1261/rna.071720.119
- [Google Scholar]
- The microbiome-mitochondrion connection: Common ancestries, common mechanisms, common goals. mSystems. 2017;2:e00018-17. https://doi.org/10.1128/mSystems.00018-17
- [Google Scholar]
- Microbe-mitochondrion crosstalk and health: An emerging paradigm. Mitochondrion. 2018;39:20-5. https://doi.org/10.1016/j.mito.2017.08.008
- [Google Scholar]
- Purine catabolism in escherichia coli and function of xanthine dehydrogenase in purine salvage. Journal of Bacteriology. 2000;182:5332-5341. https://doi.org/10.1128/JB.182.19.5332-5341.2000
- [Google Scholar]
- Uric acid in metabolic syndrome: Does uric acid have a definitive role? European Journal of Internal Medicine. 2022;103:4-12. https://doi.org/10.1016/j.ejim.2022.04.022
- [Google Scholar]
- Mitochondrial ROS govern the LPS-induced pro-inflammatory response in microglia cells by regulating MAPK and NF-κB pathways. Neuroscience Letters. 2015;584:191-196. https://doi.org/10.1016/j.neulet.2014.10.016
- [Google Scholar]
- Peroxiredoxin I is a ROS/p38 MAPK-dependent inducible antioxidant that regulates NF-κB-mediated iNOS induction and microglial activation. Journal of Neuroimmunology. 2013;259:26-36. https://doi.org/10.1016/j.jneuroim.2013.03.006
- [Google Scholar]
- The Crosstalk between the Gut Microbiota and Mitochondria during Exercise. Frontiers in Physiology. 2017;8:319. https://doi.org/10.3389/fphys.2017.00319
- [Google Scholar]
- The role of glutathione reductase and related enzymes on cellular redox homoeostasis network. Free Radical Biology and Medicine. 2016;95:27-42. https://doi.org/10.1016/j.freeradbiomed.2016.02.028
- [Google Scholar]
- Phosphate binding in PNP alters transition-state analogue affinity and subunit cooperativity. Biochemistry. 2023;62:3116-3125. https://doi.org/10.1021/acs.biochem.3c00264
- [Google Scholar]
- Isocitrate dehydrogenase gene variants in cancer and their clinical significance. Biochemical Society Transactions. 2021;49:2561-2572. https://doi.org/10.1042/BST20210277
- [Google Scholar]
- Comprehensive analysis of epigenetics regulation, prognostic and the correlation with immune infiltrates of GPX7 in adult gliomas. Scientific Reports. 2022;12:6442. https://doi.org/10.1038/s41598-022-10114-1
- [Google Scholar]
- GPX7 reduces chondrocyte inflammation and extracellular matrix degradation triggered by IL 1β, via a mechanism mediated by ferroptosis. Molecular Medicine Reports. 2024;30:118. https://doi.org/10.3892/mmr.2024.13242
- [Google Scholar]
- Molecular properties and regulation of NAD+ kinase (NADK) Redox Biology. 2023;59:102561. https://doi.org/10.1016/j.redox.2022.102561
- [Google Scholar]
- Xanthine oxidoreductase: One enzyme for multiple physiological tasks. Redox Biology. 2021;41:101882. https://doi.org/10.1016/j.redox.2021.101882
- [Google Scholar]
- The beneficial effects of traditional Chinese medicine on antioxidative status and inflammatory cytokines expression in the liver of piglets. Frontiers in Veterinary Science. 2022;9:937745. https://doi.org/10.3389/fvets.2022.937745
- [Google Scholar]
- Xiaojianzhong decoction attenuates aspirin-induced gastric mucosal injury via the PI3K/AKT/mTOR/ULK1 and AMPK/ULK1 pathways. Le Pharmacien Biologiste. 2023;61:1234-1248. https://doi.org/10.1080/13880209.2023.2243998
- [Google Scholar]
- Synergistic effects of Chinese herbal medicine: A comprehensive review of methodology and current research. Frontiers in Pharmacology. 2016;7:201. https://doi.org/10.3389/fphar.2016.00201
- [Google Scholar]
- Deciphering the mechanical network of chronic atrophic gastritis: a urinary time-dependent metabonomics-based network pharmacology study. Frontiers in Physiology. 2019;10:1004. https://doi.org/10.3389/fphys.2019.01004
- [Google Scholar]
- Study of Zuojin pill in treating chronic atrophic gastritis by UPLC-Q-TOF/MS Based on serum and urine metabolomics combined with network pharmacology. International Journal of Analytical Chemistry. 2021;2021:1-15. https://doi.org/10.1155/2021/6649600
- [Google Scholar]